Abstract
1. INTRODUCTION
According to a definition issued by the International Hydrographic Organization (IHO), Hydrography is the branch of applied sciences which deals with the measurement and description of the physical features of oceans, seas, coastal areas, lakes and rivers, as well as with the prediction of their change over time, for the primary purpose of safety of navigation and in support of all other marine activities, including economic development, security and defense, scientific research, and environmental protection. (IHO, 2022). In this context, the term bathymetry is also in use. Both words stem from Greek and while hydrography translates to “describing water”, bathymetry means ”measuring depths”. Thus, bathymetry is a more specific term, but both can be used interchangeably in the sense of mapping underwater geometry.
Precise knowledge of the shape and change of underwater topography and objects is the basis for a variety of socio-economic and ecological topics. The former include safety of navigation, flood risk assessment, hazard zone and protection planning, and the latter include restoration of coastal and alluvial areas, monitoring of their state and change, and assessment of the respective effects on aquatic habitats (hydrobiology, habitat modeling on micro- and meso-scale, etc.).
Optical methods are well suited for capturing bathymetry of clear and shallow coastal and inland water bodies with depths <60m from the air but are inappropriate for deeper waters due to the high absorption of light in water. In case the carrier platform is under water, optical methods can also be applied for mapping both the seabed and natural or human-made objects in deep water if the measuring distance is small. Hydroacoustic methods (Sound Navigation And Ranging, SONAR) are the first choice for medium water depths of 20–500m (Lurton, 2010). While SONAR systems can also measure deep water, satellite gravimetry and altimetry provide global coverage of deep ocean areas (Sandwell et al., 2014) with a spatial resolution in the km-range. Figure 1 schematically illustrates the depth categories and the appropriate hydrographic techniques.
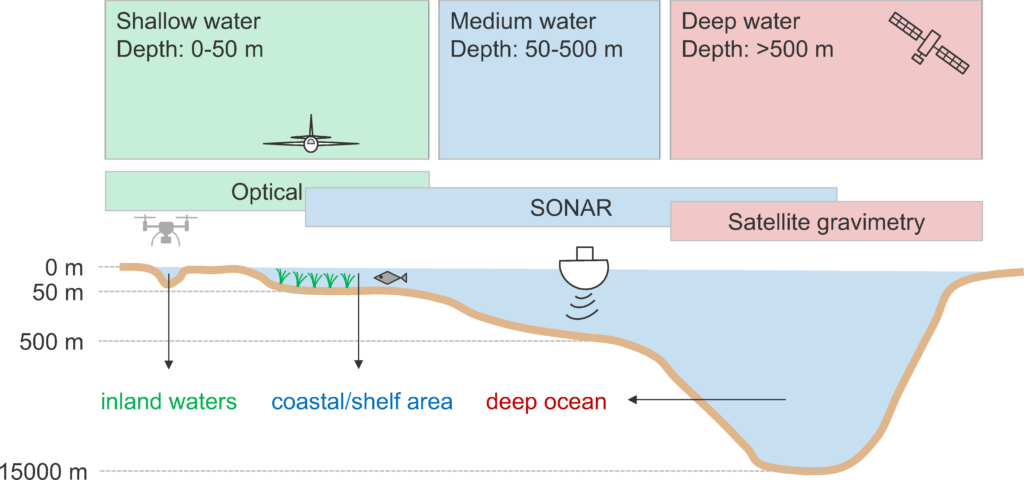
Within the shallow water domain, Figure 1 indicates an overlap between optical methods and SONAR. In fact, SONAR is the state-of-the-art capturing method for navigational purposes of both coastal and inland waterways and outperforms optical methods concerning depth penetration. However, the advantage of airborne optical methods is twofold: (i) the effective SONAR Field-of-View (FoV) drops with decreasing water depth, whereas the swath width mainly depends on the flying altitude and only to a minor extent on the water depth for airborne data acquisition and (ii) shipborne SONAR requires a minimum water depth for safe operation (cf. Figure 2). Furthermore, airborne optical methods provide a seamless coverage from the water bottom via the littoral zone to the dry nearshore area (Guenther et al., 2000; Schwarz et al., 2019; Yang, Qi et al., 2022).
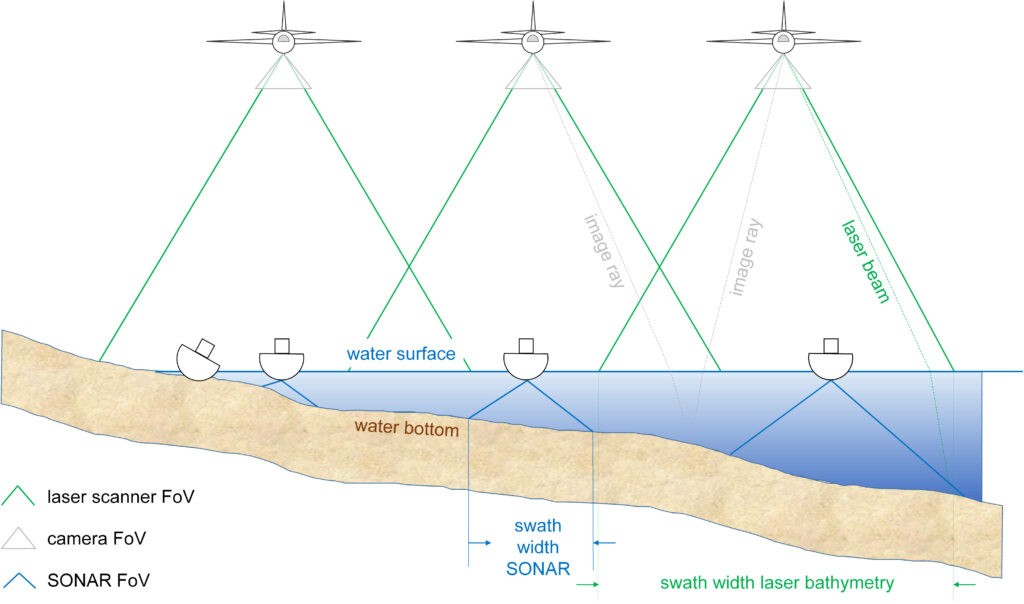
For hydrographic surveys using optical remote sensing, the following methods have become state-of-the-art: (i) spectrally derived bathymetry based on multispectral images (SDB), (ii) multimedia photogrammetry based on stereo images also referred to as photo bathymetry, and (iii) airborne laser bathymetry (ALB) also referred to as airborne laser hydrography (ALH). While the first two methods are passive and use backscattered solar radiation from the bottom of the water body for depth measurements, ALB is an active method based on Time-of-Flight (ToF) measurements of a pulsed green laser. In the past, the above mentioned methods were used separately. However, today a clear trend towards hybrid multi-sensor systems integrating both cameras and laser scanners can be observed (Fuchs and Tuell, 2010; Toschi et al., 2018; Legleiter and Harrison, 2019). Figure 3 shows a schematic diagram of the three main optical methods in hydrography.
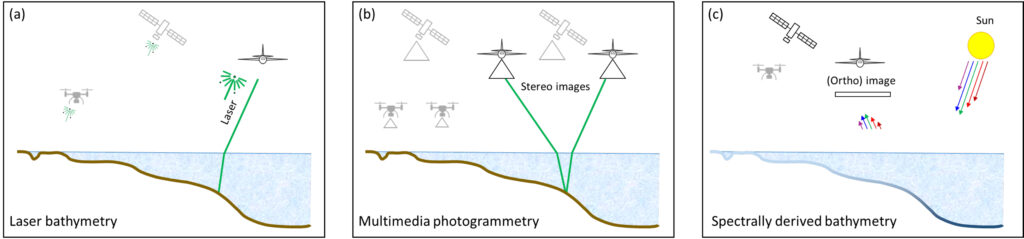
The seminal paper on multimedia photogrammetry dates back to 1948 (Rinner, 1948). The first applications of the laser (light amplification by stimulated emission of radiation) invented in the 1960s were finding sub-marines (Sorenson et al., 1966) and mapping near-shore bathymetry (Hickman and Hogg, 1969). Not much later, Poicyn et al. (1970) described multispectral approaches to derive water depth from satellite images. Thus, all the known passive and active optical hydrography methods have been introduced before 1970 and we are already looking back at 50 years of history in optical hydrography. Today’s science community can built upon a considerable amount of seminal research on subject matters and developments concerning sensors, platforms and processing strategies are still boosting the field. This can clearly be seen by the increasing number of publications in the recent years. The results of a bibliographic query in the citation database Scopus using the keywords spectrally derived bathymetry, multimedia photogrammetry and laser bathymetry (including similar terms like photo bathymetry, bathymetric LiDAR (Light Detection And Ranging), satellite bathymetry, etc.) are plotted in Figure 4.
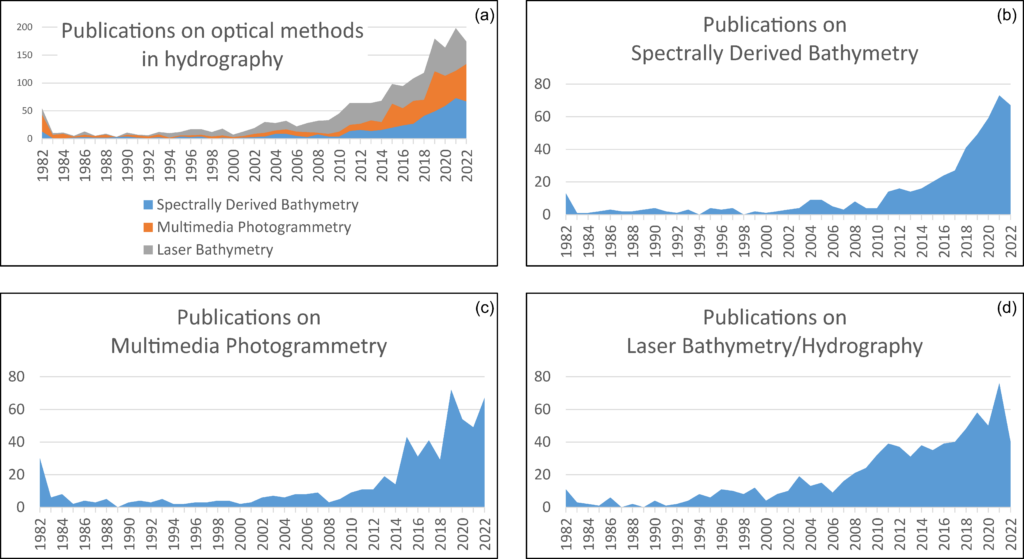
query in the Scopus citation database.
The results are presented as a summary of all optical methods (Figure 4a) and separately for SDB (Figure 4b), photo bathymetry (Figure 4c) and laser bathymetry (Figure 4d).
The small peak at the beginning of the timeline in 1982 results from aggregating earlier contributions into the first bin. Apart from that, it can clearly be seen from Figure 4a that until around the year 2000 there were less then 20 papers published per year. Following a first smaller peak around 2004 with approximately 30 articles per year, a continuous increase started from 2010 on. On a particular level, it is interesting to note that more multimedia photogrammetry articles were published in the early days (before 1982, cf. left peak in Figure 4c). The likely reason for that is the earlier availability of photographic (stereo) cameras compared to multispectral satellites and bathymetric laser scanners. For SDB, it is noted that Landsat Level-1 multispectral images as well as Level-2 and Level-3 science products have been made available for download from the U.S. Geological Survey (USGS) archive at no charge from 2008 on, which entailed a continuous rise of SDB-related publications starting in 2010 (cf. Figure 4b). For laser bathymetry (Figure 4d), a considerable peak can be seen in 2011 following the introduction of topo-bathymetric laser scanners, which aimed at providing high resolution for shallow water areas compared to the traditional low resolution scanners optimized for depth performance.
The prominent peaks in 2014 and 2019 in Figures 4c and 4d, respectively, can be related to the increasing availability of UAV-borne (uncrewed aerial vehicle) imaging sensors. The stimulating impact of UAVs as carrier platforms is a general trend for photogrammetric mapping and 3D object reconstruction (Colomina and Molina, 2014). With respect to hydrographic applications (Yang, Yu et al., 2022), it is noted that the rise came again earlier for multimedia photogrammetry, as cameras are much lighter and it took longer to develop compact and lightweight scanners. However, advances in sensor and platform development are not the only driving force in this field; increased computing power has also enabled the use of machine learning techniques in geoscience in general (Dramsch, 2020) and in optical hydrography in particular (Al Najar et al., 2021; Moran et al. 2022).
When compared to a search with the general keywords hydrography or hydrographic (not shown in Figure 4), it is striking that until the year 2010 the percentage of scientific articles on optical bathymetry is only around 10%. However, from 2010 on, the percentage rises to a level of more than 40% in 2021. This is another indication that optical methods are gaining importance in the recent past.
In general, Figure 4 reveals that within the last four decades the publication activity has increased by an order of magnitude with around 200 articles in 2020 and the trend is still unbroken as the apparent decline in 2022 is attributed to a conservative estimate for the 2nd half of 2022. Table 1 contains a selection of recent publications related to optical methods in hydrography highlighting the relevance of the topic. It can be seen from both Table 1 and Figure 4 that all three main fields (SDB, photo and laser bathymetry) are equally vital in the sense of publication activity and no distinct priority of a certain method is observable in general. However, Table 1 clearly reveals that SDB methods are mainly used with satellite images, laser bathymetry is predominantly employed from crewed aircraft, and multimedia photogrammetry is either performed from UAV or underwater platforms. The high number of publications underlines the importance of current research, especially against the background of climate change and its effects, such as the increase in flood disasters on the one hand and increasing water scarcity and droughts on the other (Kreibich et al., 2022).
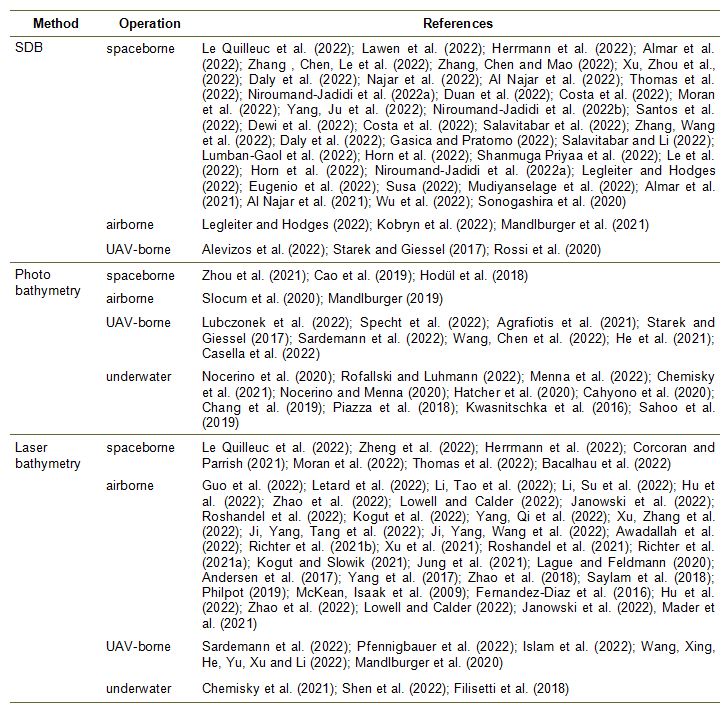
The objective of this paper is to provide an overview of methods, sensors, platforms, and applications of optical measurement techniques for hydrographic mapping, focusing on through-water techniques, i.e., applications where the sensor is located above the water surface and measurements are performed through the air-water interface. The article is structured as follows: The first part of the paper introduces the principles of the three main optical methods in Section 2 (SDB), Section 3 (photo bathymetry), and Section 4 (laser bathymetry). Each section discusses the basics of the respective method but also addresses open research areas. The second part of the paper focuses on sensors and platforms in Section 5 and presents selected applications in Section 6. The article ends with a summary and concluding remarks in Section 7.
2. SPECTRALLY DERIVED BATHYMETRY
In spectrally derived bathymetry (SDB), a relationship is established between the radiometric image content and the water depth (Poicyn et al., 1970; Lyzenga, 1978; Philpot, 1989; Maritorena et al. 1994). The prerequisite for this is a thorough understanding of the complex interaction of solar radiation with the atmosphere, the water surface, the water body and finally the bottom of the water body as a function of the wavelength λ. In general, two approaches are used for deriving bathymetry from the radiometric image content: (i) physics-based methods (cf. Section 2.1) and (ii) regression based approaches (cf. Section 2.2). The latter requires independent reference data to train models describing the color-to-depth relationship for which purpose machine learning techniques play an important role in the modern literature (cf. Section 2.3).
2.1 Physics-based approach
The total radiance arriving at the image sensor can be written as the sum of individual partial contributions (Legleiter et al., 2009):

(1)
Figure 5 illustrates Equation 1, in which the total radiation LT incident at the sensor consists of the radiation LB reflected from the bottom of the water body, the radiation LC backscattered from the water body or water column, respectively, the signal component from reflections at the water surface LS and components from backscattering particles in the atmosphere LP. Figure 5 shows the relationships schematically. The signal attenuation within the water column is exponential as a result of continuous forward and backward scattering as well as signal absorption in the water column. The signal contribution LB from the bottom depends on both water depth and bottom properties (reflectance, roughness). The bottom reflectance considerably varies for different soil types ranging, e.g., from 0.1 to 0.3 for wet limestone and from 0.03 to 0.06 for wet gravel within the entire visible spectrum of 400–800nm (Legleiter et al., 2009). However, the spectral differences of the bottom play a major role only in very shallow water, since in deeper water the attenuation by the water column predominates (Röttgers et al., 2014; Pope and Fry, 1997).
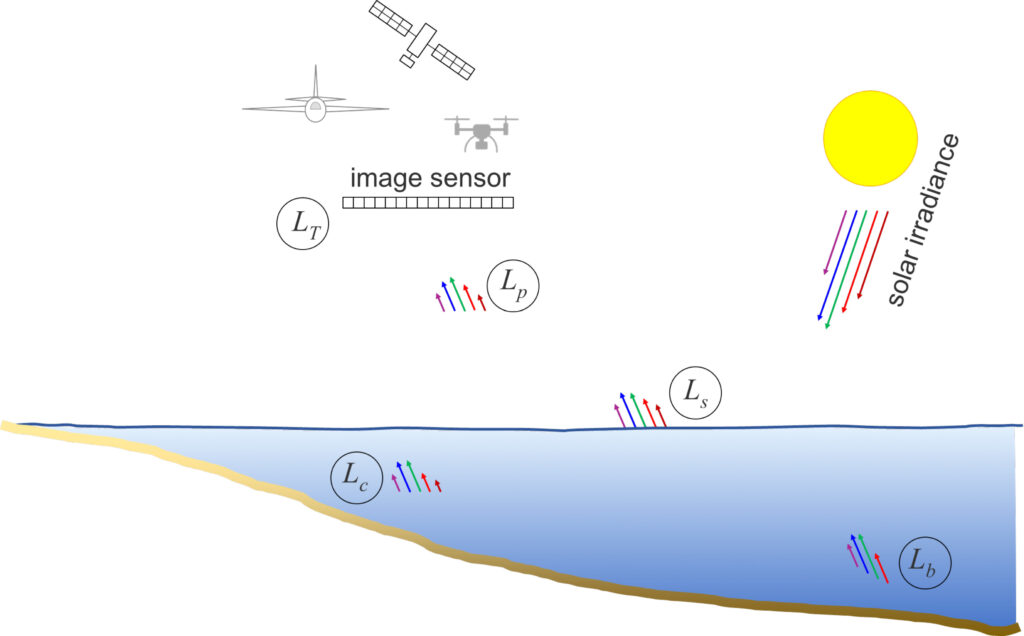
The contribution from the water column LC is determined by the water’s optical properties. The contributing factors are once more absorption and scattering by pure water, but also turbidity caused by suspended sediment and organic matter (Grobbelaar, 2009). Depending on the viewing direction, the term LS may account for a large fraction of the total signal LT due to specular reflection at the water surface. Such specular reflections occur when the sunlight is directly reflected from the water surface into the FoV of a sensor pixel. Depending on the motion of the water surface (waves), specular pixels occur either sporadically or in clusters. In any case, the corresponding image areas must be masked during data preprocessing and deactivated for further processing. To a certain degree, the influence of sun glint can be mitigated based on the infrared channels of a multispectral image as described by Kay et al. (2009). Finally, LP represents path radiance backscattered from the atmosphere into the sensor’s FoV (Zhao et al., 2012; Stumpf and Pennock, 1989).
For images containing optically deep water, a simple physical relationship between water depth and backscatter strength can be formulated (Lyzenga et al., 2006):

(2)
L(d) is the radiation received at the sensor after correction for atmosphere and any specular highlights (sun glint). The LS term in Equation 2 includes reflections from the water surface as well as backscatter from an infinitely deep water column. LB primarily describes the reflectivity of the bottom, but also includes transmission losses passing through the air-water interface and effects of volume scattering in the water body. The exponential coefficient α is the effective attenuation coefficient and consists of the sum of forward and backward scattered light components.
Equation 2 shows the exponential signal decay as a function of the water depth d and the water body’s optical properties (α) already mentioned above. By taking the antilogarithm, a linear relationship between the water depth and the radiometric image content can be established and the water depth d can be calculated directly by a simple transformation.
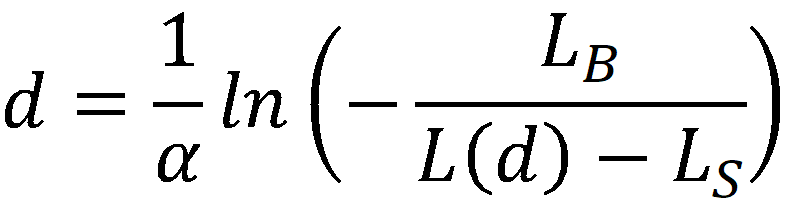
(3)
Thus, assuming that both the water conditions and the subsurface are homogeneous, the depth can already be determined from a single spectral image channel without the presence of external reference data. However, since both signal absorption in the water column and bottom reflectance depend on wavelength, in practice multiple radiometric bands of multispectral images are used to determine the unknown parameters remaining in Equation 3. This is usually done as part of an optimization task based on reference data from terrestrial or echosounder surveys (Lyzenga et al., 2006).
2.2 Regression based approach
While Equations 2 and 3 represent a physically motivated model, one of the disadvantages is that LB depends on both water depth (d) and bottom reflectance (RB) and thus both effects are interlaced. An approach to overcome this limitation was introduced in Stumpf et al. (2003) by calculating the ratio of two spectral bands with different wavelength or index, respectively, which was found to be approximately constant and thus to a certain extent independent from variations of bottom reflectance. Still it assumes the presence of optically deep regions, i.e. areas where LB is entirely dominated by the backscatter of the (endlessly deep) water column and does not contain any signal from the bottom. However, e.g. for mapping clear and moderately deep alpine rivers or shallow coastal areas like coral reefs with UAV images (Alevizos et al., 2022), this is not necessarily the case. In the absence of optically deep water, still the deepest part of the scene can replace LB at d=∞ as a pragmatic solution (Legleiter, 2016). A more generic solution is to apply the log-transformed band ratio (Stumpf et al., 2003) and to identify the optimum band combination in an approach referred to as Optimum Band Ratio Analysis (OBRA; Legleiter et al., 2009). This results in an image-derived quantity X:
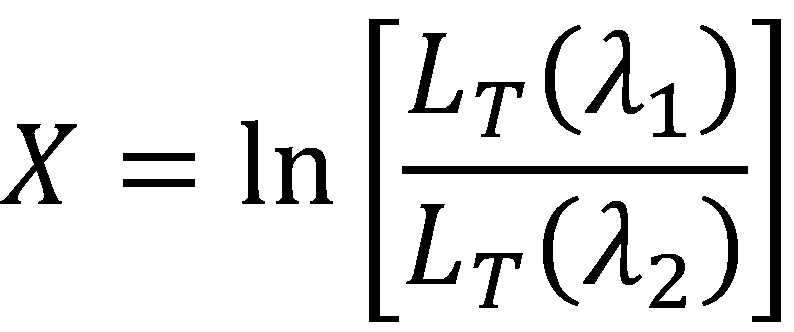
(4)
which is approximately linearly related to the water depth d. The empirical relationship between X and d is finally established by regressing reference depths from field surveys against X. A linear regression function will be sufficient, if Equation 4 sufficiently accounts for all non-linear effects, otherwise higher order functions (e.g., polynomials of degree n) can also be used, as in the example shown in Figure 6, where depth profiles of a mountain lake in Tyrol, Austria were surveyed with a compact SONAR system and an areal depth map was created via SDB using the OBRA approach based on aerial RGBI images.
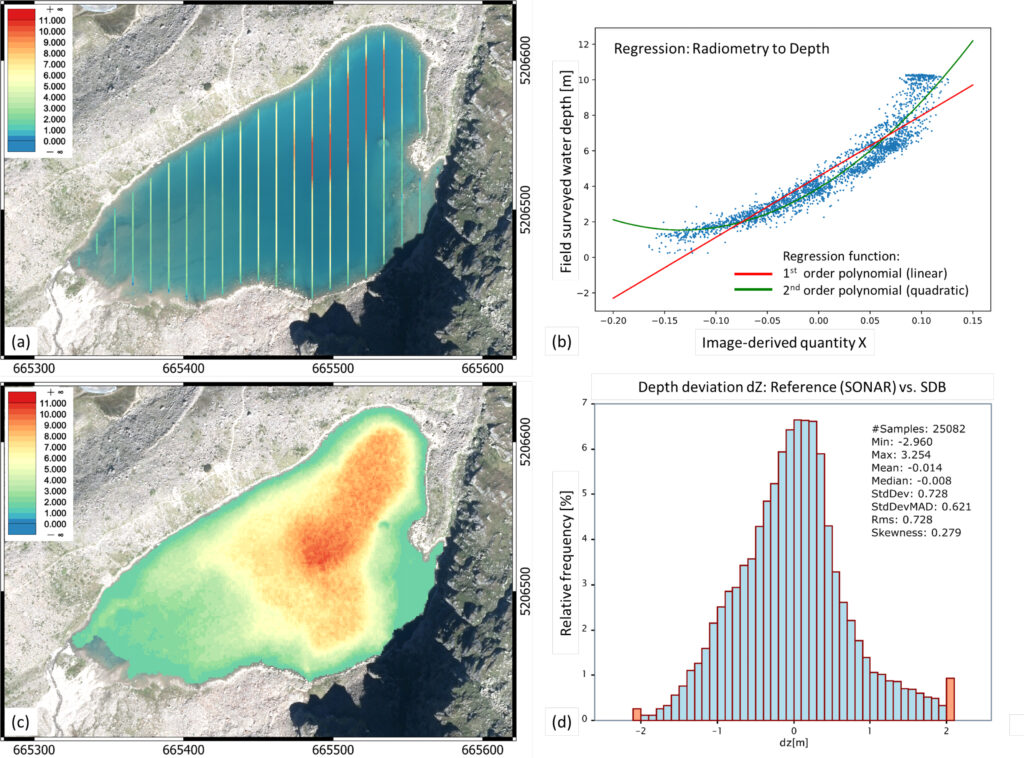
2.3 Machine learning in SDB
In addition to the well established physics- and regression-based depth inversion methods discussed above, machine learning (ML) approaches have gained increasing attention for various geoscience applications in general (Dramsch, 2020) and SDB in particular (Al Najar et al., 2021) in the recent decade. While a core strength of ML is classification, especially convolutional neural networks (CNN) have proven to be effective depth predictors due to ability of convolutions to approximate arbitrary functions (Zhou, 2020; Murtagh, 1991). This is particularly important in the context of SDB because the interaction of light with the water surface, water column, and water bottom is complex, and simple models that focus on hand-crafted features such as specific log-transformed band ratios may not be able to capture the full complexity of spectral image information.
Among the variety of classical ML approaches, simple artificial neural networks (Makboul et al., 2017), k-nearest neighbor regression (Legleiter and Harrison, 2019), random forest (Sagawa et al., 2019; Yang, Ju et al., 2022), gradient boost (Susa, 2022), multilayer perceptrons (Duan et al., 2022), back propagation neural networks (Wu et al., 2022), ensemble learning (Eugenio et al., 2022) and support vector machines (Misra et al., 2018) have been successfully applied for deriving bathymetry from multispectral images. Beyond that, a current trend towards deep neural networks can be observed. The employed methods include simple neural networks (Niroumand-Jadidi et al., 2022a,b), locally adaptive back-propagation neural networks (Liu et al., 2018), recurrent neural networks (Dickens and Armstrong, 2019), deep learning based image super-resolution (Sonogashira et al., 2020), and CNNs (Al Najar et al., 2021; Mandlburger et al., 2021; Lumban-Gaol et al., 2022; Ji, Yang, Tang et al., 2022; Mudiyanselage et al., 2022). It is noted that the application field is not restricted to coastal areas but also includes inland waters like rivers and lakes (Legleiter and Harrison, 2019; Mandlburger et al., 2021).
3. MULTIMEDIA PHOTOGRAMMETRY
A complementary image-based method for depth determination is multimedia photogrammetry. This is a purely geometric method, the fundamentals of which date back to the mid of the 20th century (Rinner, 1948). The technique is applied to capture the underwater topography of hydrodynamic lab facilities based on images from terrestrial or crane-mounted cameras, but is also used to survey rivers based on crewed and uncrewed aerial images or even high-resolution stereo satellite images. The fundamentals are reviewed in the following subsections.
3.1 Two-media case
With the advent of digital photogrammetry (Förstner and Wrobel, 2016) and automated evaluation methods from the field of Computer Vision like Structure from Motion (SfM) (Schonberger and Frahm, 2016) and Dense Image Matching (DIM; Hirschmuller, 2008; Haala and Rothermel, 2012; Rothermel et al., 2012; Wenzel et al., 2013), the topic of photogrammetric depth determination for capturing topographic data from stereo images has received increased attention. This also applies to multimedia photogrammetry. In case the sensor is in the air or on ground and the surveyed objects and surfaces are submerged, this results in a two-media problem also referred to as through-water photogrammetry.
Modern literature discusses stereo image-based acquisition of underwater topography for running waters (Butler et al., 2002; Westaway et al., 2001; Dietrich, 2016) and for coastal areas based on stereo images captured with drones, crewed aircraft and satellites (Hodül et al., 2018; Cao et al., 2019; Agrafiotis et al., 2020; Wang, Chen, et al., 2022).
Building on the basic concept of photogrammetry (Kraus, 2007; Förstner and Wrobel, 2016), underwater topography can be derived from stereo images provided that the inner and outer orientation of the images is known (Mulsow, 2010) and the water surface can be reconstructed with sufficient accuracy (Zou et al. ,2016; Engelen et al., 2018). Image orientation is facilitated in the through-water case if the water body is small and does not cover the entire image area. If enough tie points are available on dry ground, standard techniques for image orientation (Förstner and Wrobel, 2016; Kraus 2007) can be used. In all other cases, a strict consideration of the refraction effect is required not only for generating dense underwater point clouds, but also for matching and reconstruction of tie points used for image orientation (Maas, 2015; Mulsow, 2010). Once the interior and exterior orientation of the images are resolved and homologous points of the water bottom can be identified in at least two images, the apparent intersection of the corresponding image rays still needs to be corrected for refraction at the water surface (Luhmann et al., 2019). The basis for this is Snell’s law of refraction:
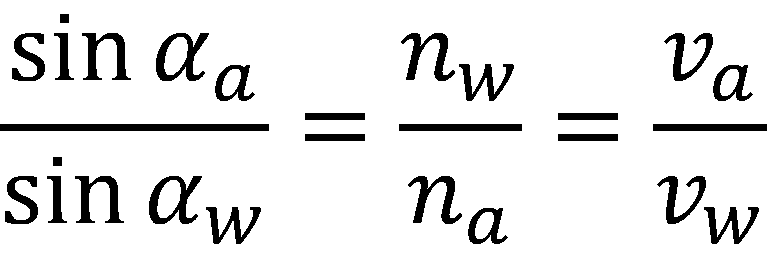
(5)
Equation 5 reveals that the sines of the air- and water-side image rays (αa and αw) are inversely proportional to the respective refractive indices in water (nw≈1.33) and air (na≈1.00) and directly proportional to the propagation velocities (va≈300.000km/s, vw≈225.564km/s). Figure 7 shows the refraction of the image rays toward the perpendicular during the transition from the optically thinner medium of air to the optically denser medium of water. As a result, the apparent image point P’, which results from the straight-line intersection of the image rays, tends to be too high and must be corrected downward by applying the refraction correction to the actual point P at the bottom of the water. Detailed descriptions of refraction correction can be found in Kotowski (1988) and Murase et al. (2008). With respect to bundle block adjustment, Maas (2015) and Kahmen et al. (2020) specify formulae to explicitly integrate refractive interfaces into the photogrammetric processing pipeline.
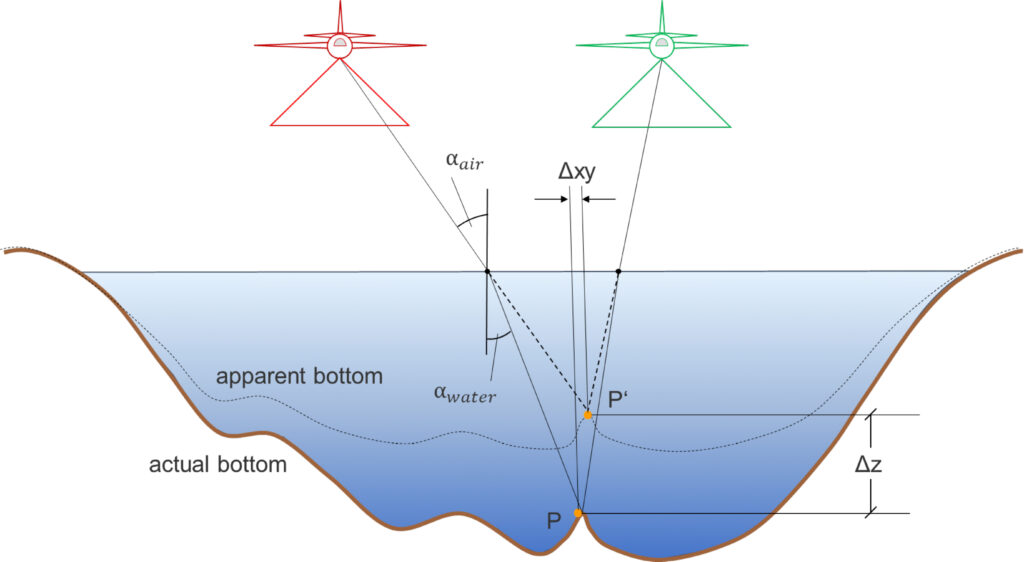
3.2 Multiple refractive interfaces
While the water surface is the only refractive interface in the simple two-media case, multiple refractive interfaces may be present in other situations. This applies to through-water photo bathymetry in case there are multiple layers with different refractive indices within the water body, but also when the sensor is underwater and the camera is sheltered against water ingress by a surrounding housing using either flat or dome ports. In the latter case, the material of the housing exhibits a certain thickness depending on the pressure it has to withstand where the image rays are refracted both when entering and exiting the shield (cf. Figure 8).
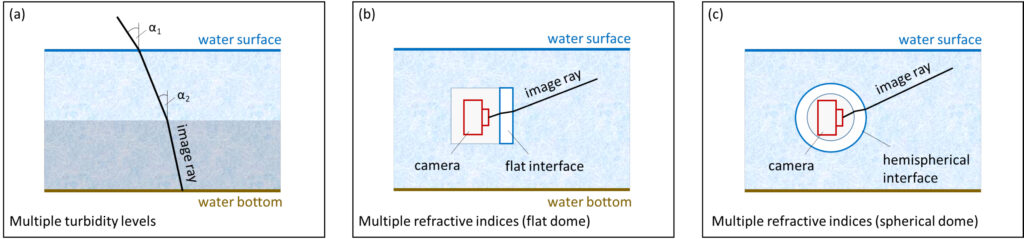
Maas (2015) describes a rigorous geometric correction model for the multi-media case, which is generic enough to serve as the basis for integration into standard photogrammetric bundle block adjustment software. This paper also discusses the accuracy potential of multimedia photogrammetry and highlights the impact of image network geometry, interface planarity, refractive index variations and dispersion as well as diffusion effects under water resulting in an accuracy degradation of about a factor two under relatively favorable conditions.
Menna et al. (2016) investigate the use of consumer grade cameras in combination with hemispherical dome ports for deriving accurate underwater 3D point clouds. The same authors published a review on flat and dome ports, discuss their pros and cons and conclude that hemispherical dome ports outperform the simpler flat ports both with respect to the residuals in image space as well as concerning precision and accuracy of the derived 3D object points (Menna et al., 2017a). It is noted that theoretically, if the camera’s projection center is located exactly in the center of a hemispherical dome and all image rays are perfectly radial, no refraction would occur at the dome’s interface. However, as the projections center is located behind the lens system of the camera, refraction also occurs when using hemispherical domes (cf. Figure 8c).
3.3 SfM and Dense Image Matching
With the advent of Computer Vision-based techniques like SfM (Schonberger and Frahm, 2016) and Dense Image Matching (Hirschmuller, 2008) in modern digital photogrammetry (Förstner and Wrobel, 2016), many photogrammetric software solutions surfaced implementing the full processing pipeline from image orientation and camera calibration, via the creation of 3D point clouds to final model derivation (3D meshes, Digital Surface Models). The most prominent commercial products are Metashape (Agisoft, 2022), Pix4D Mapper (Pix4D, 2022), the Match-T/
Match-AT/OrthoMaster/UAS-Master software suite (Trimble, 2022a), CapturingReality (Trimble, 2022c), PhotoModeller (Trimble, 2022b) and SURE (nFrames, 2022) as well as their open source counterparts like MicMac (Rupnik et al., 2017), Bundler (Bundler, 2022), and VisualSFM (Wu, 2022), to mention just a few. All these products can generally also be used for photo bathymetry (Burns and Delparte, 2017).
In general, two major steps can be identified in any photogrammetric processing pipeline: (i) image orientation and camera calibration and (ii) point cloud generation via feature based, area based or dense matching approaches. The prior (i.e. image orientation) has already been discussed in Section 3.1. Concerning dense surface reconstruction, the derivation of topographic point clouds can be considered mature, but there are still open research questions in photo bathymetry (Mandlburger, 2019). This especially holds for non-static water surfaces in the through-water case. While in the topographic case, more stereo image partners generally increase the accuracy of the resulting point cloud, direction-dependent image ray refraction can possibly lead to a deterioration of the results in photo bathymetry. The problem of varying refraction effects for every point/camera combination in a SfM point cloud is also addressed in Dietrich (2016). The author presents a multi-camera refraction correction and reports accuracy in the order of 0.1% of the flying altitude, i.e., 4cm at a flying altitude of 40m above ground level. Starek and Giessel (2017) propose a denoising method to filter underwater SfM points and combine multimedia photogrammetry and spectral depth inversion to generate seamless underwater models. For the underwater case, the topic of dense object reconstruction is discussed by Hatcher et al. (2020) for mapping coral reefs using a towed surface vehicle with an onboard survey-grade Global Navigation Satellite System (GNSS) and five rigidly mounted downward-looking cameras with overlapping views of the seafloor providing sub-centimeter resolution.
3.4 Machine learning in photo bathymetry
It already became clear in Section 2.3 that ML approaches play an important role in SDB. This is much less the case for multimedia photogrammetry as the technique is purely geometric and for most problems like pose estimation and point cloud generation deterministic approaches exist. However, the difficulty to capture and model the dynamic water surface in sufficient temporal and spatial resolution opens the floor for applying ML-based techniques for refraction correction. Agrafiotis et al. (2019) introduced a support vector regression (SVR) model (DepthLearn), which was trained using reference depths captured with bathymetric LiDAR. The authors showed that the SVR model can successfully compensate the systematic underestimation of the raw SfM-derived water depths. In a follow-up work, Agrafiotis et al. (2020) apply the DepthLearn model to correct refraction in image-space. With the resulting refraction-free images, dense image matching pipelines based on multi-view stereo delivers unbiased bathymetric maps. The accuracy of the ML-based refraction correction could be further improved by using synthetic data for model training (Agrafiotis et al., 2021). This was shown for aerial images from both crewed and uncrewed platforms.
4. LASER BATHYMETRY
In contrast to the passive methods described in Sections 2 and 3, laser bathymetry in general and airborne laser bathymetry (ALB) in particular represents an active technique for mapping shallow waters using a pulsed green laser (Philpot, 2019; Guenther et al., 2000). The basics and selected specific aspects of laser bathymetry are detailed in the following subsections.
4.1 ALB basics
In ALB, the distance between sensor and target is determined by measuring the round-trip travel time of a very short laser pulse (wavelength λ = 532nm, pulse duration ∆t=1–10ns) through air and water (Guenther et al., 2000). The measurement process is schematically sketched in Figure 9.
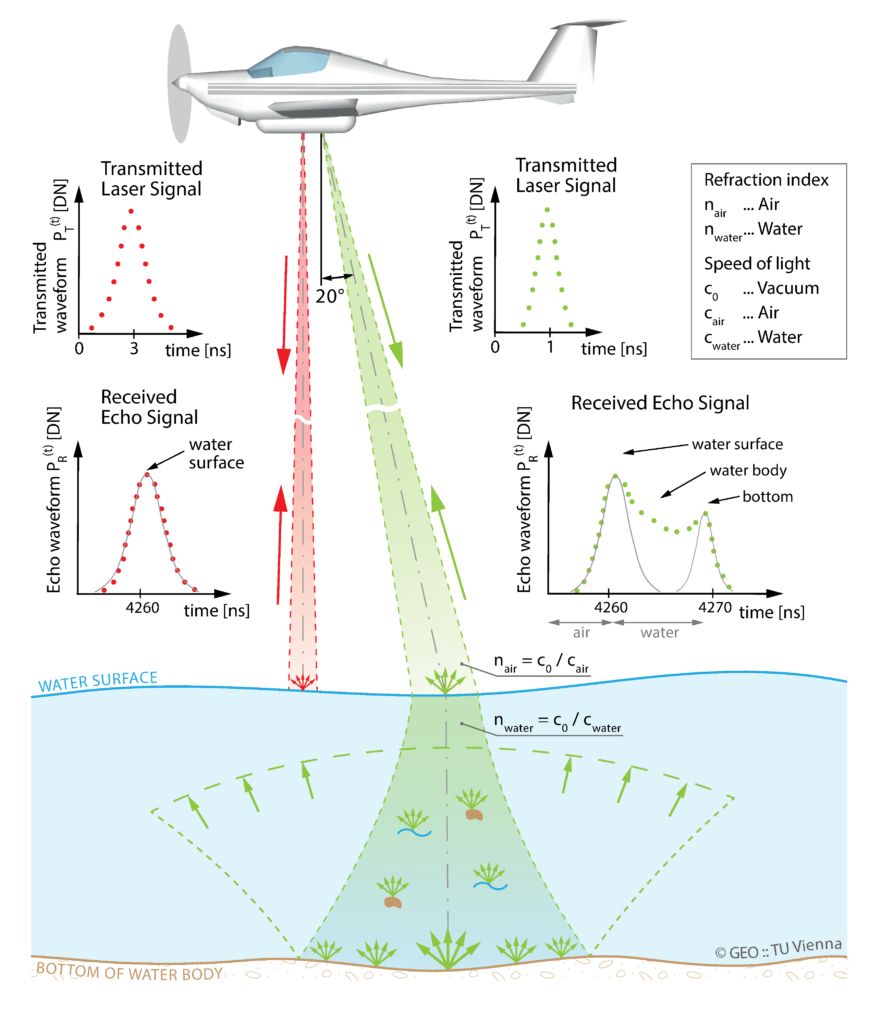
After pulse emission and traveling through the atmosphere, the laser beam is partially reflected at the water surface and the remaining part penetrates into the water body. Upon entering the water column, the laser beam changes both its direction depending on the waters’ optical properties and its propagation speed according to Snell’s law of refraction (cf. Equation 5, vW≈vL/1.33≈225.564km/s). Due to the lower speed of light in water, the uncorrected 3D underwater measurement points appear too deep in contrast to multimedia photogrammetry, and must be corrected upward accordingly (cf. Figure 10).
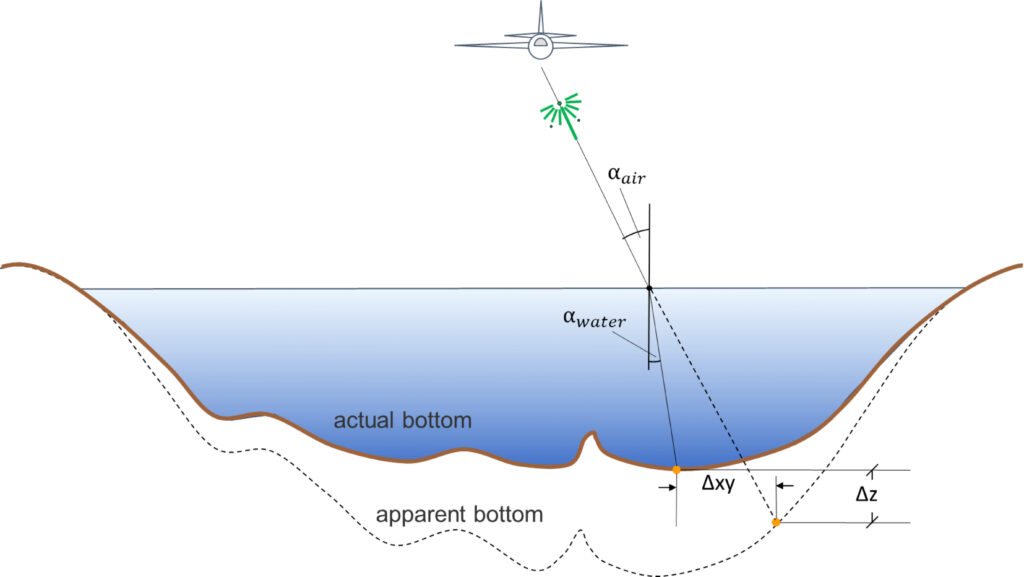
In the water column, the laser radiation is attenuated by continuous beam refraction and signal absorption, so that after reflection of the laser pulse at the bottom and the corresponding return path, only a small part of the laser energy arrives at the sensor. Thus, all bathymetric sensors employ very sensitive detectors (Quadros, 2013; Mandlburger, 2020). The general relationship between transmitted and received energy is described by the laser-radar equation (Wagner, 2010), which for bathymetric applications is divided into the signal components from the water surface, the water column, the bottom of the water body, and background radiation including losses in the atmosphere (Abdallah et al., 2012; Tulldahl and Steinvall, 2004).

(6)
Equation 6 has the same form as Equation 1. The signal losses in laser bathymetry are thus equivalent to those already described in Section 2 for SDB. This also holds for the exponential attenuation in the water column. A key advantage of laser bathymetry is that the signal attenuation, usually described by the effective attenuation coefficient k, can be estimated from the asymmetric shape of the recorded waveforms (Richter et al., 2017; Schwarz et al., 2017). There is a direct relationship between the attenuation coefficient k and the Secchi depth sd (sd≈1.6/k). The Secchi depth is an empirical measure for water turbidity and refers to the distance beyond which the black and white quadrants of a 20cm-diameter disk released into the water from a boat on a rope can no longer be distinguished from each other (Effler, 1988). The manufacturers of bathymetric sensors usually describe the depth measurement performance in multiples of the Secchi depth (Quadros, 2013).
4.2 Water surface detection and refraction correction
The detection of the water surface is a prerequisite for precise refraction and run-time correction of the raw measurements. Most bathymetric scanners operate an additional near infrared channel (λ=1.064nm) together with the green laser for this purpose, since signal absorption in water is very high for near-infrared (NIR) radiation, and it therefore penetrates only minimally into the water column (Guenther et al., 2000; Ewing, 1965). If no NIR channel is present, the air-water interface needs to be modeled from the green channel reflections alone. Since in this case, the echoes from the water surface often represent a mixture of direct reflection and volume scattering in the first cm of the water column (Guenther et al., 2000), special evaluation and modeling methods are required. Especially for topo-bathymetric scanners with small laser footprint, the non-planarity and dynamics of the water surface (waves) have to be considered to obtain precise 3D point coordinates of the water bottom (Westfeld et al., 2017).
The employed approaches include statistical methods, which aggregate neighboring (near) surface echoes to define the water level height (Mandlburger et al., 2013; Mandlburger and Jutzi, 2019), methods based on clustering with connectivity constraints (Roshandel et al., 2021), and strict mathematical modeling of the entire underwater laser path resulting in water surface height estimates for each laser pulse (Schwarz et al., 2019). Next to detecting distinct surface echoes in the laser measurements, it is equally important to reconstruct the water surface as a continuous surface providing both elevation and slope at arbitrary positions. For this purpose, raster models (Mandlburger et al., 2013), triangular irregular networks (Ullrich and Pfennigbauer, 2012) or free-form surfaces (Richter et al., 2021a) are in use.
Once the water surface is reconstructed, refraction and signal run-time correction can be performed. In the modern literature, Xu et al. (2021) present a method for strict correction of sea surface wave-induced refraction errors for each bottom point by calculating the water surface normal directions for each laser pulse entering the water body. To calculate the air-side angle of incidence αa used in Equation 5, both the known direction vector of the laser beam and the normal direction of the water surface are required. From this, the resulting water-side angle can then be calculated if the refractive indices of air and water (na, nw) are known. A similar approach, also considering water surface slopes, is proposed by Yang et al. (2017) based on sea surface profiles and ray tracing. Schwarz et al. (2021) highlight the difference of phase and group velocity, which was long neglected in laser bathymetry. While the phase velocity (i.e., speed of laser light @532nm) is decisive for beam deflection, the group velocity (i.e., speed of laser pulse) must be used for the ToF correction.
All the mentioned aspects as well as sensor orientation and calibration contribute to the total error budget of laser bathymetry. Applying rigorous error propagation, Eren et al. (2019) published a model for estimating the Total Vertical Uncertainty (TVU) of bathymetric LiDAR.
4.3 Full waveform analysis
Unlike topographic laser scanning, where simple time-to-digital converters can be used to measure the round-trip time of a laser pulse (Ullrich and Pfennigbauer, 2016), bathymetric LiDAR requires digitization of the entire backscatter waveform, referred to as full waveform (FWF), due to the complex interaction of the laser pulse with the water medium (Guenther et al., 2000). FWF processing can either be done online during the flight (Pfennigbauer and Ullrich, 2010; Pfennigbauer et al., 2014) or off-line, if the waveforms are additionally stored on hard disk. Comparisons of different techniques for processing laser bathymetry waveforms were published by Wang et al. (2015) and Allouis et al. (2010). The standard methods include peak detection, average square difference function, Gaussian decomposition, quadrilateral fitting, Richardson–Lucy deconvolution, and Wiener filter deconvolution. In addition, Schwarz et al. (2017) introduced a physically motivated approach referred to as exponential decomposition. The approach was later narrowed down to typical bathymetric mapping situations with signal interaction at the surface, volume and bottom (Schwarz et al., 2019). This explicit model also addresses the fundamental problem of resolving very shallow water depths, where the returned signal peaks from surface and bottom overlap. This allows to measure ultra shallow water depths and provides a strictly seamless water-land transition. The topic of resolving very shallow water depths is also addressed in Yang, Qi et al. (2022), where a signal resolution enhancement model and a fractional differentiation mathematical tool are used together with Gaussian decomposition (Wagner et al., 2006) to measure water depths of less then 10cm.
An inherent problem in laser bathymetry is the restricted depth penetration capability due to (i) the general attenuation in water and (ii) water turbidity caused by floating or suspended sediment. Kogut and Bakula (2019) analyzed neighboring return waveforms to identify missing bottom points based on the prior knowledge of existing bottom points in the vicinity. To explicitly enhance the signal-to-noise ratio (SNR), Mader et al. (2022) propose a non-linear full waveform stacking technique. The authors first average neighboring return waveforms and subsequently identify surface and bottom points in the original waveforms within a restricted search corridor. With this method, an extra 30% of depth penetration is achieved without smoothing the resulting point clouds. Stacking and other full waveform processing methods are also reported in Steinbacher et al. (2021) along with the implementation in a bathymetric software suite.
4.4 Laser triangulation
Most of the bathymetric laser scanners operate based on the ToF measurement principle, i.e., the round-trip time of a laser pulse is measured and converted in to a distance. Especially for underwater close-range application, laser lightsheet triangulation is an alternative to ToF scanning. To operate laser triangulation in a hydrographic context, a green laser line is projected onto the object and the illuminated line or curve, respectively, is captured by a camera mounted at a fixed base with respect to the laser projector (Sardemann et al., 2022). The imaging system is installed inside a watertight housing and the sensors need to be placed oblique within the housing to obtain near-orthogonal ray intersection angles. Refraction effects at the air-glass interface inside and the glass-water interface outside the housing need to be considered to obtain precise 3D object coordinates.
One of the main advantages over ToF-based laser bathymetry is that highly accurate underwater measurements can be achieved at much lower costs. In the recent past, different implementations of bathymetric scanners based on the lightsheet triangulation principle have been described (Bleier et al., 2019; Xue et al., 2021; Lee et al., 2021), all of which feature sub-mm precision for a limited depth range of less than 50 cm. An exhaustive review of underwater scanners including but not limited to triangulation based scanners can be found in (Castillón, et al. 2019).
4.5 Hybrid methods
While multimedia photogrammetry and laser bathymetry constitute self-contained methods, SDB relies on external reference data for model calibration. For this purpose, SONAR data are often used for water areas beyond a wadeable depth of about 1.5m and otherwise terrestrial surveys (GNSS, total station). The advent of hybrid sensor systems combining laser scanners and cameras on the same platform now make it possible to use remote sensing based techniques for generating the reference data for training and calibration of respective SDB models. This specifically holds for crewed and uncrewed aerial platforms (Mandlburger, 2020). However, also satellite-based techniques benefit from the availability of spaceborne LiDAR sensors with bathymetric capabilities (Parrish et al., 2019).
Recent publications therefore use hybrid processing pipelines. Ji, Yang, Tang et al. (2022) use ALB point clouds for precise feature-based registration (orientation) of satellite images. Combinations of photo bathymetry and spectral depth estimation are published in Slocum et al. (2020) and Starek and Giessel (2017) based on aerial images only. Mandlburger et al. (2021) use the water surface and bottom models derived from topo-bathymetric LiDAR as reference for training, testing and validating a bathymetric CNN (BathyNet) to derive bathymetry from concurrently captured multispectral images (RGB+coastal blue). Such a trained network can potentially be used later for camera-only surveys. An example, where UAV-based photo bathymetry was used to train depth inversion models for satellite images is presented in Wang, Chen et al. (2022).
On a global scale, the combination of spaceborne LiDAR, specifically from the Advanced Topographic Laser Altimeter System (ATLAS) aboard ICESat-2 (Markus et al., 2017) and multispectral satellite images from Sentinel-2, Landsat-8, WorldView-2, Pleiades, etc. is increasingly used for SDB solely relying on remote sensing data. Examples for using ATALS data for calibration of spectral depth inversion models are published in Thomas et al. (2022), Le et al. (2022), Zhang, Chen, Le et al. (2022), Herrmann et al. (2022), Hartmann et al. (2021), Cao et al. (2021) and Le Quilleuc et al. (2022).
5. SENSORS AND PLATFORMS
Optical hydrographic methods are employed both on global and local scale. The measurement range varies from 800km to a few centimeters. The used sensor platforms are either operated from spaceborne, crewed or uncrewed airborne, terrestrial or underwater platforms. In the underwater case, the sensors are either carried by divers, remotely operated vehicles (ROV) or autonomous underwater vehicles (AUV). An overview of the platforms and scales used in optical hydrography is provided in Chemisky et al. (2021). The following subsections introduce a representative selection of sensors and platforms starting with satellite sensors (Section 5.1) via airborne sensors based on crewed (Section 5.2) and uncrewed (Section 5.3) platforms, to underwater sensors (Section 5.4).
5.1 Space-borne sensors
On a global scale, multispectral satellite images constitute the primary source for deriving hydrographic maps of the shallow water zone. Table 2 provides a list of frequently used multispectral satellites for both spaceborne multimedia photogrammetry and spectrally derived bathymetry.
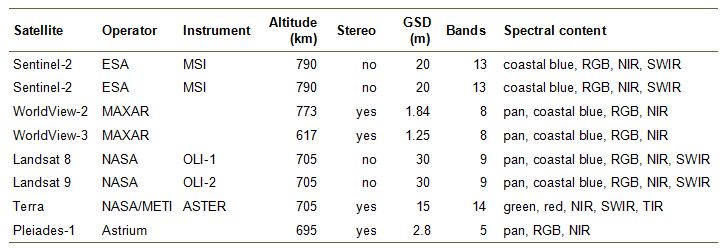
The GSD reported in Table 2 refers to the multispectral bands. In addition, most sensors also provide a panchromatic channel often at higher spatial resolution. The pan channels are beneficial for photo bathymetry but do generally not add extra information for SDB. The provided spectral bands typically include a water-penetrating coastal blue channel in the ultra-violet domain of the spectrum (λ≈440nm), multiple visible channels (blue, green, red, red edge) as well as near infrared (NIR), short wave infrared (SWIR), and thermal infrared (TIR) channels. For deriving hydrographic products, the NIR channels provide the basis for sun glint corrections (Lyzenga et al., 2006) and the visible channels are employed for deriving bathymetry.
SDB based on multispectral satellite images constitute by far the most often employed use case. Reasons for the popularity include the open data access of some products (e.g., Sentinel-2, Landsat 8 etc.), the maturity of the technique, the narrow image FoV of typically much less then ±10° which spares refraction correction, and the fact that single images are a sufficient data basis. In the recent literature, applications have been reported in Le Quilleuc et al. (2022), Lawen et al. (2022), Herrmann et al. (2022), Almar et al. (2022), Zhang, Chen, Le et al. (2022), Zhang, Chen and Mao (2022), Xu, Zhou et al. (2022), Daly et al. (2022), Najar et al. (2022), Al Najar et al. (2022), Thomas et al. (2022), Niroumand-Jadidi et al. (2022a), Mudiyanselage et al. (2022), Almar et al. (2021), Al Najar et al. (2021), Wu et al. (2022), and Sonogashira et al. (2020).
In contrast to that, stereo images are required for multimedia photogrammetry, which narrows down the potential satellite choice (e.g. WorldView, Pleiades, Terra/ASTER). Still, also an increased interest can be observed, as this purely geometric technique is self-contained and does not require external reference data. Applications are, e.g., reported in Hodül et al. (2018) and Cao et al. (2019).
While the derivation of hydrographic products from spaceborne platforms has long been restricted to passive images, the advent of ICESat and its successor ICESat-2 (Markus et al., 2017; Neumann et al., 2019) changed this situation fundamentally. While the ATLAS aboard ICESat-2 does not provide full areal shallow water coverage at high spatial resolution, it perfectly complements existing multispectral instruments by providing reliable underwater reference topography on a per laser spot basis. This is especially useful for deep learning based SDB approaches which require abundant training data. This apparent advantage has already been used by several researchers (cf. Section 4.5).
ATLAS is a single-photon sensitive laser altimeter using green laser radiation (λ=532nm), thus, ideally suited for bathymetric purposes next to its prime application of capturing the Earth’s cryosphere. The LiDAR sensor contains six transmitters and corresponding receivers, which are aligned in three parallel lines. Three high energy lasers (pulse energy: 1.2mJ) are always paired with a corresponding low energy laser (strong:weak beam energy ratio=4:1). The pulse repetition frequency is 10kHz, which results in an along-track point spacing of 0.7m with a laser footprint diameter of 14m (i.e., along-track oversampling). The across-track spacing of the corresponding strong-weak laser pairs measures 90m and the spacing of the three pairs amounts to 3.3km (i.e. across-track undersampling). The revisit cycle is 91 days, thus enabling multi-temporal applications on a global scale. A thorough description and assessment of the bathymetric capabilities of ATLAS is published in Parrish et al. (2019). An spaceborne oceanographic LiDAR simulator is presented in Zhang, Chen and Mao (2022) highlighting that next to the commonly used green laser wavelength of 532nm, the use of lasers in the coastal blue and blue domain of the spectrum (λ=440/490nm) would achieve the greatest depth in oligotrophic seawater in the subtropical zone.
5.2 Airborne sensors
The classical application of optical hydrography is from crewed aircraft. All acquisition methods discussed in Sections 2–4 are employed from crewed airborne platforms. Any kind of metric camera, which is conventionally used for topographic applications and orthophoto production, can also be used for deriving hydrographic products. This especially applies to photogrammetric cameras providing a NIR channel alongside with the visible channels. Examples include the UltraCam camera series (Vecxel imaging) or the MFC150 camera (Leica Geosystems). Today, medium format cameras manufactured by PhaseOne are playing an increasingly important role due to their light weight and flexible integration options. The disadvantage of not featuring a 4-band RGBI product can be compensated by integrating two cameras with different spectral filters (Mandlburger et al., 2021).
Airborne laser bathymetry sensors can be divided into: (i) deep bathymetric, (ii) shallow topo-bathymetric, and (iii) multi-purpose sensors. Deep bathymetric sensors aim at maximizing the penetration depth. They employ lasers with relatively long pulse duration of around 7ns and low measurement rate of 3–10kHz to achieve high pulse energy of approx. 7mJ. To comply with eye safety regulations, the beam divergence of such sensors is large (7mrad) resulting in a laser footprint diameter of 3–4m when operated at an altitude of 500m. Thus, a high depth penetration of typically 3sd comes at the prize of a moderate spatial resolution.
The so-called topo-bathymetric sensors focus on higher spatial resolution for capturing shallow inland and coastal water areas with high relief energy (rocks, boulders, sudden slope changes, etc.). They use short and narrow laser beams (pulse duration: 1–2ns, beam divergence: 0.7–2mrad) and higher pulse repetition rates of up to 700kHz resulting in laser footprint diameters of 0.5–1m on the ground and a point density of around 25 points/m2 in a single flight strip. The short pulse length enables separation of laser returns from water surface and bottom also for very shallow areas with water depths less than 20cm and thus a seamless transition between water and land. On the other hand, short pulse lengths also entail a lower pulse energy and consequently a lower depth penetration of typically 1.5sd.
In the recent years, multispectral and Single Photon LiDAR (SPL) scanners were introduced in the market. Both types of instruments are not specifically tailored for hydrographic mapping but, nevertheless, exhibit bathymetric capabilities (Fernandez-Diaz et al., 2016; Degnan, 2016).
Airborne multispectral laser scanners feature all three commonly used laser wavelengths (λ=532/1064/1550nm) and enable the derivation of vegetation indices facilitating point classification (Fernandez-Diaz et al., 2016). The main purpose of SPL is wide-area topographic mapping (Degnan, 2016), but due to the use of (i) a green laser and (ii) very sensitive detectors, this technology also comes with moderate bathymetric capabilities with a depth penetration performance of approx. 1sd. It is noted that Single Photon LiDAR technology is also used by the ATLAS instrument aboard the ICESat-2 satellite (Markus et al., 2017; Neumann et al., 2019; Parrish et al., 2019).
Traditional ALB systems utilize coaxial infrared and green laser beams for water surface and water bottom detection on a per pulse basis (Guenther et al., 2000; Wozencraft and Lillycrop, 2003; Fuchs and Tuell 2010). Other sensors use disjoint infrared and green lasers (cf. Figure 9). Such a design allows precise reconstruction of static water surfaces from the non-water penetrating infrared channel. Some modern ALB instruments, however, use green lasers only to detect both water surface and bottom (Wright et al., 2016; Pfennigbauer et al., 2011). This poses challenges for water surface modeling as the laser return signal from the water surface comprise intermingled components of specular reflections at the air-water interface and sub-surface volume backscattering. The derivation of precise water surface models from green-only scanners thus requires sophisticated data processing (Thomas and Guenther, 1990; Birkebak et al., 2018a,b; Schwarz et al., 2019).
Table 3 summarizes the specs of selected ALB scanner systems. The list contains examples for both deep and shallow topo-bathymetric sensors. The first instrument (LADS HD+) features a deep bathy channel only and the following two (HawkEye 4X, CZMIL SuperNova) contain both deep and shallow bathy channels. In all three cases, the parameters of the deep bathy channels are listed. The remaining instruments all constitute topo-bathymetric scanners containing one shallow water and an additional IR channel (Chiroptera-5, VQ-880-GH) or two shallow water channels (EAARL-B; McKean, Nagel et al., 2009). For these instruments the specs of the shallow bathy channels are reported. Except for the pulse repetition rate, the specs of topographic IR channels are not contained in Table 3. All reported values have been collected to the best of the author’s knowledge based on the manufacturer’s spec sheets and/or published papers.
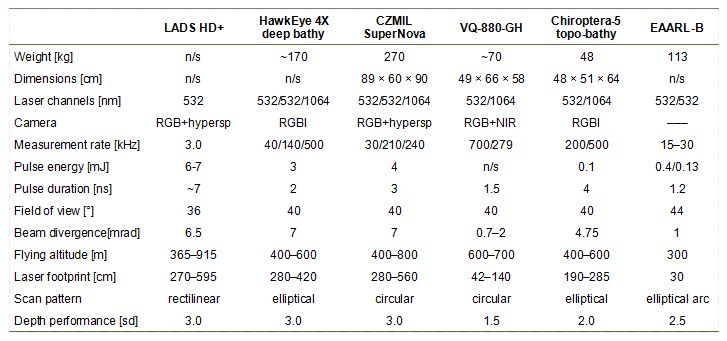
Table 3 illustrate that the deep bathy sensors provide a high penetration depth of up to 3.0sd but moderate measurement rate of 3–40kHz resulting in a point density of ≤2 points/m2 and large footprint sizes in the range of 3–7m. The topo-bathymetric scanners, in turn, feature small footprint diameters in the sub-m range, higher measurement rates of up to 700kHz at the price of limited depth penetration (1.5sd). In addition to the laser scanners, most of the listed sensors also contain RGB or RGBI cameras. The images are mainly employed for photo documentation or as data basis for point cloud colorization, but the use of high-resolution metric cameras (e.g., RCD30, PhaseOne IXU, etc.) also opens the floor for bathymetry estimation via both photogrammetry or SDB. Respective use cases for using concurrently captured bathymetric LiDAR and multispectral images for SDB model calibration have already been discussed in Section 4.5.
Concerning vertical accuracy, all sensors listed in Table 3 meet one of the accuracy standards formulated by the International Hydrographic Organization (IHO, 2020). Especially the topo-bathymetric instruments are designed to comply to the rigorous versions of the standard like the Special Order specification requiring a Total Vertical Uncertainty (TVU) of 25cm for 95% of the measured bottom points and a Total Horizontal Uncertainty (THU) of 2m along with a 100% bathymetric coverage.
5.3 UAV-borne sensors
Until a few years ago, bathymetric laser scanners could only be operated from crewed platforms (aircraft, helicopters, gyrocopters) due to their considerable weight (cf. Table 3). With ongoing sensor miniaturization and progress in the development of uncrewed aerial platforms, compact laser scanners can now also be integrated on both fixed-wing and multi-rotor UAVs. Drones are typically operated from low flying altitude of about 50–120m above ground level and with moderate flying velocity of 4–10m/s entailing a significantly smaller laser footprint size as well as a higher point density and, thus, a higher spatial resolution compared to operation from crewed airborne platforms at higher altitudes. Furthermore, due to the shorter measurement range, signal attenuation in the atmosphere is also significantly lower and more signal strength is effectively available for penetrating the water body. This especially applies to UAV-borne bathymetric laser sensors but also plays a role for image derived bathymetry.
As light-weight cameras were available long before the advent of compact laser scanners, the use of UAV-cameras for photogrammetric mapping in general (Colomina and Molina, 2014) and hydrographic application in particular (Dietrich, 2016) have emerged earlier than UAV-borne laser bathymetry. As already stated in Section 5.2, all cameras which are suited for mapping topography are also suited for hydrography. While high-end camera systems including an IR channel in addition to the visible RGB are often available for crewed airborne platforms, this is rarely the case for UAV-images. However, ongoing research demonstrates that RGB images are an appropriate basis for both multimedia photogrammetry and SDB (He et al., 2021; Templin et al., 2018; Carrivick and Smith, 2019; Watanabe and Kawahara, 2016; Gentile et al., 2016; Shintani and Fonstad, 2017; Dietrich, 2016; Koutalakis and Zaimes, 2022; Rossi et al., 2020; Wang, Chen et al., 2022; Specht et al., 2022; Alevizos et al. 2022).
In most cases, multi-rotor UAV platforms are used for image-based hydrography with the distinct advantage of the versatility of such platforms (i.e., minimal space for starting/landing required, stop-and-go mode, arbitrary waypoint-based flight paths). In addition to that, fixed-wing UAVs are also in use (He et al., 2021; Escobar Villanueva et al., 2019; Templin et al., 2018). They feature longer flight endurance and therefore higher areal coverage. In general, the growing mass market for UAVs with image and video capabilities boosts the use of such consumer-grade instruments for hydrographic applications. A prominent example is the DJI Phantom 4 RTK drone featuring a 20 MPix RGB camera with a mechanical (global) shutter. While the Phantom 4 camera is not metric in the strict sense, the inner orientation is sufficiently stable to allow the derivation of 3D point clouds above and below the water table.
In the recent past, the advent of bathymetric laser scanners integrated on UAVs with a maximum take-off mass (MTOM) below 35kg can be seen as another major leap in the field of airborne laser bathymetry with respect to spatial resolution as well as depth performance. Just as the advent of shallow-water topo-bathymetric scanners in addition to traditional deep-water sensors has increased spatial resolution, UAV-based topo-bathymetric scanners have boosted achievable point density by another order of magnitude. The laser footprint diameter of modern UAV-borne bathymetric scanners is in the sub-dm range, and together with point densities in the order of 100–200 points/m2 this not only enables mapping of submerged topography in high details but also allows detection and modeling of flow-relevant micro-structures like small boulders.
To date, only a few UAV-borne bathymetric laser scanners are available. The ASTRALiTe sensor (Mitchell and Thayer, 2014) is a scanning polarizing LiDAR. The sensor uses a 30mW laser, is typically operated from low flying altitude of around 20m above ground level and therefore provides limited areal measurement performance. Also the depth performance of 1.2sd is moderate, but the small weight allows integration on many commercially available multi-rotor UAV platforms. The RAMMS (Rapid Airborne Multibeam Mapping System, (Mitchell, 2019; Ventura, 2020) features a remarkable depth penetration of 3sd and strictly avoids moving parts. With every laser shot, the sensor emits an entire laser line, which is captured by multiple receivers. The concept therefore resembles the principle of multibeam echo sounding with a single ping and multiple transducers. The instrument weighs 14kg and is rather designed for integration on light aircraft and helicopters but can also be mounted on powerful UAV platforms. The same also applies to the VQ-840-GL (weight: 10kg), where beam deflection is realized with a rotating mirror. The scanner features a user definable beam divergence and receiver’s FoV allowing to balance depth measurement performance (≥2sd) and spatial resolution (Mandlburger et al., 2020). The main parameters of the described instruments are summarized in Table 4.
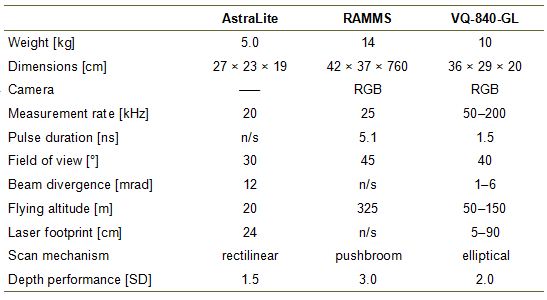
5.4 Underwater sensors
Although the article mainly focuses on optical hydrographic methods, where the sensor is located above the water table, the following section contains a brief discussion of underwater sensors and platforms. In general, the four different scenarios schematically sketched in Figure 11 can be distinguished: (a) the vessel is floating on the water surface and the imaging sensors (cameras and/or laser scanners) are located at the bottom of the vessel within a watertight housing, (b) a scuba diver is manually operating a single camera or a stereo camera rig, (c) imaging sensors are integrated on a remotely operated vehicle (ROV) with a wire-based communication link, and (d) imaging sensors are installed on an autonomous underwater vehicle (AUV).
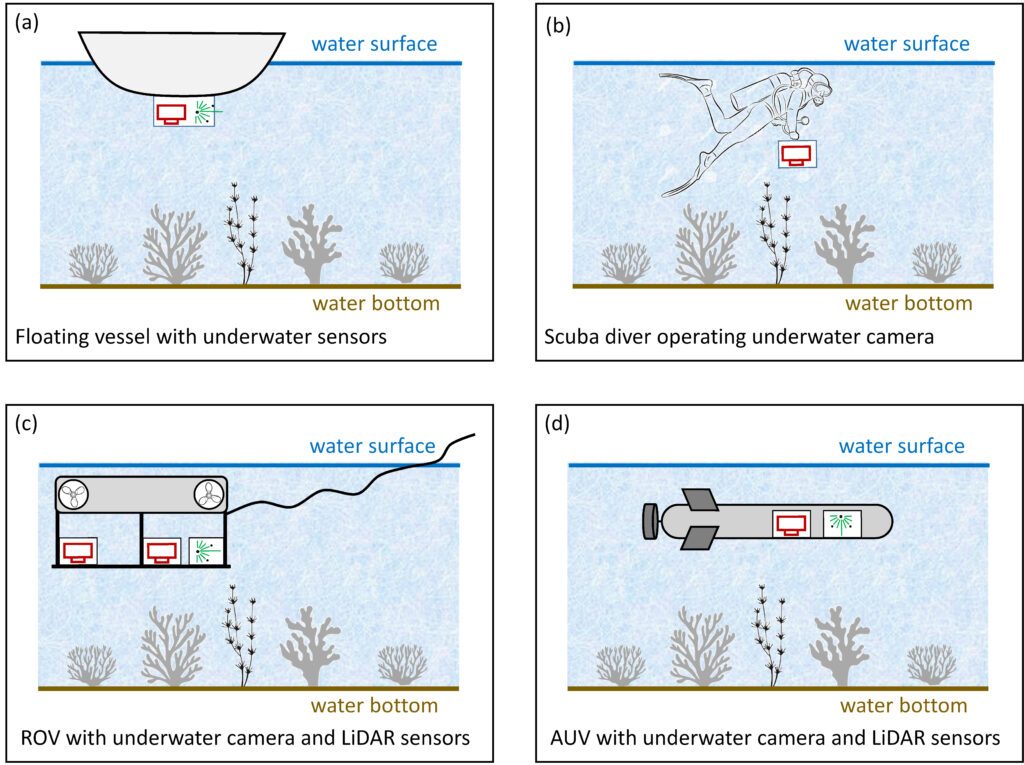
In any case, the distance between sensor and target is relatively small, which allows mapping objects in very high spatial resolution but poses additional challenges for sensor orientation. Accept for the floating vessel case, not only the sensor but also the platform is entirely under water, thus, GNSS is not available for positioning the sensor. In the GNSS-denied case, image orientation is accomplished either via control points (Maas 2015, Cahyono et al. 2020), visual odometry (Botelho et al., 2010), or Simultaneous Localization and Mapping (SLAM) techniques (Barkby et al., 2009; Massot-Campos et al. 2016; Ma et al., 2020). Under water, imaging sensors can either be handheld by a scuba diver or mounted on a ROV or AUV, respectively. The use of ROVs for underwater inspection is becoming increasingly widespread with applications in mapping and monitoring of off-shore facilities and hydro-power plants. A review of inspection-based ROVs is published in Capocci et al. (2017). Besides ROVs, fully autonomously operating underwater vehicles are also rapidly developing. They are already used for mapping large areas of the seafloor in depths of several thousand meters. In an early review, Bellingham (2009) describes the principle of operation and navigation of AUV platforms. Considering the absence of GNSS in underwater areas, localization and navigation mainly relies on inertial navigation and SLAM (Sahoo et al., 2019). Next to hydroacoustic sensors, AUVs also integrate optical imaging sensors like lasers and stereo cameras, but application is hampered among other things by the absence of light and by constraints concerning energy consumption. Despite these difficulties, high resolution mapping was already successfully conducted for square kilometers of deep ocean floor (Kwasnitschka et al., 2016).
While spectral methods are rarely used in underwater photogrammetry, the application of SfM-based photo bathymetry is widespread. Both mirrorless cameras (Sony a1, Sony a7R, Olympus E-PL10, Nikon Z7, Canon EOS R5, etc.) as well as digital single-lens reflex (DSLR) cameras (Canon EOS Rebel SL3, Nikon D850/780/500) are in use. In any case, the cameras need to be operated within a waterproof housing, for which purpose flat and dome ports can be employed. The differences between both housings concepts are analyzed and discussed in Menna et al. (2017b,a).
Next to stereo-photogrammetry, laser scanning is also used under water. Due to eye safety, scanners are predominantly integrated on ROVs and AUVs. Underwater laser scanners are operated using (i) the ToF measurement principle based on pulsed green lasers, (ii) triangulation based on structured light, and (iii) frequency modulation. More detailed reviews of under water laser scanning can be found in Filisetti et al. (2018) and Massot-Campos and Oliver-Codina (2015).
6. APPLICATIONS
The applications of SDB, photo and laser bathymetry are manifold and more use case scenarios are currently emerging due to the tremendous progress in sensor and platform technology. Especially sensor miniaturization and the introduction of remotely piloted or even autonomously operating platforms open new possibilities for mapping, inspection, monitoring and documentation of underwater topography, artifacts, and infrastructure. It is beyond the scope of this paper to address all application fields, but exemplary use cases are discussed in the following subsections.
6.1 Large-area shallow water mapping
Optical methods are well suited for mapping shallow water areas with moderate depths of less than 60m. The most effective technique for large-area mapping is satellite derived bathymetry. Daly et al. (2022), for example, report about mapping of a 4000km stretch along the West African coast based on Sentinel-2 multispectral images up to a depth of 35m also featuring details like ebb delta lobes and underwater dunes. A global approach of satellite-based coastal bathymetry was published in Almar et al. (2021). The authors claim that the seafloor could be resolved up to depth of 100 m covering most continental shelves with an area of 4.9 million km2. While the depth accuracy of 6–9m is moderate, the global coverage is of particular interest for countries which do not have the possibility to carry out in-situ measurements.
Next to SDB, also airborne laser bathymetry based on crewed aircraft can provide large-area coverage with a much higher vertical accuracy complying with strict IHO standards (IHO, 2020). Many reports are available highlighting the potential of ALB for wide-area mapping with meter resolution and sub-meter vertical accuracy for coastal areas, e.g., in the Baltic Sea (Song et al., 2015; Ellmer, 2016) to name just one example. The widespread use of bathymetric LiDAR is also documented by the availability of (open) data archives, e.g. managed and maintained by NOAA (National Oceanic and Atmospheric Administration) in the U.S.A. (NOAA, 2022).
6.2 Ship navigation
For navigational purposes, high positional and vertical accuracy as well as full areal coverage is required. In general, satellite based techniques based on multispectral images do not fulfill the stringent IHO requirements and airborne laser bathymetry is therefore the method of choice for nautical applications where safety and ease of navigation are paramount. Precise nautical charts are an indispensable prerequisite to enable safe ship navigation in both coastal environments (Wozencraft and Millar, 2005) as well as for navigable inland rivers. For the latter, high spatial resolution is required in addition to the high accuracy requirements, which is why topo-bathymetric laser scanners are the first choice. An application example at the Elbe River in Germany is published in Kühne (2021) based on methods and software described in Steinbacher et al. (2021).
6.3 Archaeology and cultural heritage
With the rise of the sea level, many archaeological sites are now submerged. This especially applies to the Roman age, where traces (ancient harbors, etc.) are found in the Mediterranean Sea. For 3D reconstruction of submerged structures, relatively high spatial resolution is necessary, for which reason topo-bathymetric LiDAR and multimedia stereo-photogrammetry are the preferred techniques. Doneus et al. (2013, 2015), for example, used laser bathymetry to record the remnants of a Roman villa in Adriatic sea in Coratia and to map traces of a late Neolithic dwelling in an Austrian freshwater lake. If higher spatial resolution than the dm level is required, close-range underwater photogrammetry is the method of choice. Drap (2012) published a book chapter related to the application of underwater photogrammetry in archaeology. Furthermore, the fusion of photogrammetric datasets from both above and below the water surface is described in Nocerino and Menna (2020) using the Costa Concordia shipwreck as a prominent example.
6.4 Coral reef mapping and monitoring
Another parade application of underwater photogrammetry is mapping of coral reefs. Corals have a very complex 3D structure that can usually only be fully captured using close-range techniques. Since coral reefs are very fragile ecosystems, monitoring their growth is an important issue in the context of climate change. Today, coral reef mapping is typically done with SfM-based methods (Cahyono et al., 2020) with stereo or multi-camera rigs carried by scuba divers (Nocerino et al., 2020). Photogrammetric data processing is often based on standard SfM software (Burns and Delparte, 2017). If the highest resolution and accuracy are not required, through-water photobathymetry using UAVs as carrier platforms can also be used (Casella et al., 2022). As an alternative to multimedia photogrammetry, airborne laser bathymetry is also suitable for mapping coral reefs (Wilson et al., 2019) with UAV-based bathymetric LiDAR being best (Wilson et al., 2019; Wang, Xing et al., 2022).
6.5 Coastal protection and monitoring
With more than 200 million people living along coastlines that are less than 5m above sea level, there is an obvious need for mapping coastal areas with a focus on protecting this sensitive transition zone between sea and land. The methods of choice are SDB when global coverage and frequent updates are more important than high spatial resolution. In the latter case, laser bathymetry is widely used and some countries even introduced mapping programs for coastal protection and monitoring at the federal level with regular update cycles, e.g., Schleswig-Holstein in Germany (Christiansen, 2016, 2021). In the U.S.A., coastal change monitoring has been conducted for decades using the Compact Hydrography Airborne Rapid Total Survey (CHARTS) system, which includes bathymetric and topographic laser scanners and aerial cameras (Macon, 2009).
6.6 Benthic habitat mapping
Another important application of optical methods in hydrography in addition to charting the bottom topography is mapping benthic habitats. For this purpose all three techniques (SDB, photo bathymetry, laser bathymetry) are suited and employed. For example, Wedding et al. (2008) used laser bathymetry to estimate substrate rugosity, which proved to be a good predictor of fish biomass. High resolution topo-bathymetric LiDAR was the prime data source used in Parrish et al. (2016) to map sea grass and estimate the impact of hurricane Sandy. Sea grass mapping based on topo-bathymetric LiDAR was also the focus in Letard et al. (2021). Mandlburger et al. (2015) investigated the use of topo-bathymetric LiDAR to map instream micro- and mesohabitats of a near natural river and their changes in response to flood events. In a recent study, Letard et al. (2022) use green and IR laser data to map and classify estuarine habitats and produced 3D maps of 21 land and marine cover types at very high resolution.
High resolution WorldView-2 satellite images served as basis for mapping sea grass in Su and Gibeaut (2013). Based on multispectral satellite images, Salavitabar and Li (2022) were able to provide a high resolution data basis for the restoration of fish habitats, and Legleiter and Hodges (2022) used multispectral aerial and satellite images to map algal density variations in shallow, clear-flowing rivers using the band ratio algorithm (Legleiter et al., 2009). Finally, various applications of SfM based on close-range UAV images for mapping fluvial habitats are described in Carrivick and Smith (2019).
6.7 Post-disaster documentation
Optical methods have proven successful in documenting the effects of disasters such as hurricanes, tsunamis, and floods. On a global scale, and when rapid response is required, satellite imagery is best because of the regular review cycle. However, the morphological changes triggered by disasters are often small-scale and therefore require high-resolution techniques such as photo bathymetry or laser bathymetry. Topo-bathymetric LiDAR was used, for example, to assess the impact of hurricane Sandy on benthic habitats (Parrish et al., 2016) and to estimate the impact of a 30-years flood event on fish habitats at a pre-Alpine gravel bed river (Mandlburger et al., 2015). In addition to airborne laser bathymetry, various UAV-based remote sensing techniques can be used for marine monitoring, including disaster documentation (Yang, Yu et al., 2022). The use of small UAVs as carrier platforms has the disadvantage that only moderate area coverage is achieved due to limited flight time. However, the versatility and low mobilization costs are clear advantages that enable rapid production of disaster maps with a high level of detail.
6.8 Infrastructure mapping and inspection
With the increase in offshore installations (oil and gas, wind turbines, etc.), the inspection, mapping and monitoring of underwater infrastructure is becoming increasingly important. The same is true for hydro-power plants. For accessing the survey objects, ROVs as well as AUVs are used (Capocci et al., 2017). Close-range multimedia photogrammetry is commonly used for the task of precise underwater infrastructure mapping as detailed in Chemisky et al. (2021). However, next to stereo cameras, also different types of laser scanners are employed (Filisetti et al., 2018; Massot-Campos and Oliver-Codina, 2015). In general, different approaches are required for infrastructure inspection and monitoring depending on the application, as sometimes very high sub-mm precision is required to check the shape of a turbine blade, for example, while in other cases it may only be necessary to check for the presence of an obstruction (i.e., classifying an image or image sequence).
7. SUMMARY AND CONCLUSIONS
This article provided an overview of optical methods in hydrography and a discussion of the sensors, platforms and typical applications. The established methods are (i) spectrally derived bathymetry, (ii) multimedia stereo photogrammetry, and (iii) laser bathymetry. All three methods can be operated from space-based, crewed and uncrewed airborne, and underwater platforms.
The spectral method is predominantly used based on multispectral satellite images with the inherent advantage of providing global coverage. For this method, external reference data for calibre-ting the physics-or regression-based models are necessary. Today, machine learning techniques increasingly replace traditional depth inversion methods.
Crewed aircraft is the platform of choice for operating laser bathymetry. This active remote sen-sing technique provides good depth penetration of about three times Secchi depth, efficient area coverage with the intrinsic advantage that swath width does not depend on water depth but only on flight altitude, and excellent position and height uncertainty that even meets the stringent specifications of the International Hydrographic Organization. The latter especially applies to the shallow water channels of modern topo-bathymetric laser scanners, which provide high spatial resolution in the sub-meter domain and a depth precision in the dm-range at the prize of a reduced depth performance. Thanks to advances in sensor and platform technology, bathymetric laser scanners can now be integrated onto UAVs, providing sub-dm spatial resolution and accuracy.
Multimedia photogrammetry, on the other hand, is mostly used in underwater surveying, i.e. both the objects and the sensor are located below the water surface. In this case, the sensors are located in waterproof housings that are either flat or spherical. Of all optical methods, multimedia photogrammetry has the longest history, dating back to a seminal work in 1948. Today, methodological development is driven by Computer Vision, which led to the introduction of Structure from Motion (SfM) and Dense Image Matching (DIM) in stereo photogrammetry in general and multimedia photogrammetry in particular.
Optical methods are limited in terms of measuring distance due to the strong light attenuation in the medium water. In addition to the pure clear water attenuation, turbidity further hampers depth penetration. The maximum achievable depth is around 60–75m in very clear water. Therefore optical methods are limited to shallow water when captured from air or space, or to use cases where the sensor is underwater and close to the object. As mentioned earlier, echo sounding is less efficient in shallow waters and operation is even dangerous in very shallow waters compared to aerial optical methods. Thus, optical and hydroacoustic methods do not compete with each other so much as they are synergistic.
A bibliographic review has shown that the number of publications in the field of spectrally derived, photo and laser hydrography has increased by an order of magnitude over the past decade and has now reached a level of around 200 publications per year. One of the reasons for this is the increasing availability of open data. This especially applies to space-borne images from multispectral satellites like Sentinel-2 or Landsat 9 and space-borne laser data from the ATLAS instrument aboard ICESat-2. Another reason is the rise of machine learning, which is about to become a standard tool for processing active and passive imaging data for hydrographic purposes. A third reason is the advent of low-cost UAVs as carrier platforms for local airborne hydrographic surveys. Finally, the availability of consumer-grade yet affordable sensors has further invigorated the field of both through-water and underwater data acquisition.
In summary, optical methods are an efficient alternative to traditional hydroacoustic surveys in shallow waters. Both techniques complement each other with regard to their respective fields of application. For the future of optical methods in hydrography, it is foreseeable that continuous advances in sensor and platform technology on the one hand and advances in processing methods and computer performance on the other will further improve the quality of the derived products and also open up new fields of research. Especially in times of climate change, multi-temporal analyses will play an increasing role. This is already well established for space-based data with corresponding data archives, but needs to be extended to local high-resolution data from airborne, UAV-based and underwater platforms.
8. REFERENCES
Abdallah, H., Baghdadi, N., Bailly, J.-S., Pastol, Y. and Fabre, F. (2012). Wa-LiD: A New LiDAR Simulator for Waters. IEEE Geoscience and Remote Sensing Letters Geosci. Remote Sensing Lett. 9(4), pp. 744–748. http://dx.doi.org/10.1109/LGRS.2011.2180506
Agisoft (2022). Metashape – Photogrammetric processing of digital images and 3D spatial data generation. http://www.agisoft.com
Agrafiotis, P., Karantzalos, K., Georgopoulos, A. and Skarlatos, D. (2020). Correcting Image Refraction: Towards Accurate Aerial Image-Based Bathymetry Mapping in Shallow Waters. Remote Sensing 12(2). https://www.mdpi.com/2072-4292/12/2/322
Agrafiotis, P., Karantzalos, K., Georgopoulos, A. and Skarlatos, D. (2021). Learning from Synthetic Data: En-hancing Refraction Correction Accuracy for Airborne Image-Based Bathymetric Mapping of Shallow Coastal Waters. PFG – Journal of Photogrammetry, Remote Sensing and Geoinformation Science 89(2), pp. 91–109. https://doi.org/10.1007/s41064-021-00144-1
Agrafiotis, P., Skarlatos, D., Georgopoulos, A. and Karantzalos, K. (2019). DepthLearn: Learning to Correct the Refraction on Point Clouds Derived from Aerial Imagery for Accurate Dense Shallow Water Bathymetry Based on SVMs-Fusion with LiDAR Point Clouds. Remote Sensing 11(19). https://www.mdpi.com/2072-4292/11/19/2225
Al Najar, M., El Bennioui, Y., Thoumyre, G., Almar, R., Bergsma, E. W. J., Benshila, R., Delvit, J.-M. and Wilson, D. G. (2022). A combined color and wave-based approach to satellite derived bathymetry using deep learning. The International Archives of the Photogrammetry, Remote Sensing and Spatial Information Sciences XLIII-B3-2022, 9–16. https://www.int-arch-photogramm-remote-sens-spatial-inf-sci.net/XLIII-B3-2022/9/2022/
Al Najar, M., Thoumyre, G., Bergsma, E. W. J., Almar, R., Benshila, R. and Wilson, D. G. (2021). Satellite derived bathymetry using deep learning. Machine Learning. https://doi.org/10.1007/s10994-021-05977-w
Alevizos, E., Oikonomou, D., Argyriou, A. V. and Alexakis, D. D. (2022). Fusion of Drone-Based RGB and Multi-Spectral Imagery for Shallow Water Bathymetry Inversion. Remote Sensing 14(5). https://www.mdpi.com/2072-4292/14/5/1127
Allouis, T., Bailly, J.-S., Pastol, Y. and Le Roux, C. (2010). Comparison of LiDAR waveform processing methods for very shallow water bathymetry using Raman, near-infrared and green signals. Earth Surface Processes and Landforms 35(6), pp. 640–650. https://onlinelibrary.wiley.com/doi/abs/10.1002/esp.1959
Almar, R., Bergsma, E. W. J., Brodie, K. L., Bak, A. S., Artigues, S., Lemai-Chenevier, S., Cesbron, G. and Delvit, J.-M. (2022). Coastal Topo-Bathymetry from a Single-Pass Satellite Video: Insights in Space-Videos for Coastal Monitoring at Duck Beach (NC, USA). Remote Sensing 14(7). https://www.mdpi.com/2072-4292/14/7/1529
Almar, R., Bergsma, E. W. J., Thoumyre, G., Baba, M. W., Cesbron, G., Daly, C., Garlan, T. and Lifermann, A. (2021). Global Satellite-Based Coastal Bathymetry from Waves. Remote Sensing 13(22). https://www.mdpi.com/2072-4292/13/22/4628
Andersen, M. S., Gergely, A., Al-Hamdani, Z., Steinbacher, F., Larsen, L. R. and Ernstsen, V. B. (2017). Pro-cessing and performance of topobathymetric LiDAR data for geomorphometric and morphological classification in a high-energy tidal environment. Hydrology and Earth System Sciences 21(1), pp. 43–63. https://hess.copernicus.org/articles/21/43/2017/
Awadallah, M. O. M., Juárez, A. and Alfredsen, K. (2022). Comparison between topographic and bathymetric LiDAR terrain models in flood inundation estimations. Remote Sensing 14(1). https://www.mdpi.com/2072-4292/14/1/227
Bacalhau, J. R., Ribeiro Neto, A., Crétaux, J.-F., Bergé-Nguyen, M. and Moreira, D. M. (2022). Bathymetry of reservoirs using altimetric data associated to optical images. Advances in Space Research 69(11), pp. 4098–4110. https://www.sciencedirect.com/science/article/pii/S0273117722001971
Barkby, S., Williams, S., Pizarro, O. and Jakuba, M. (2009). An efficient approach to bathymetric slam. 2009 IEEE/RSJ International Conference on Intelligent Robots and Systems. pp. 219–224.
Bellingham, J. (2009). Platforms: Autonomous underwater vehicles. J. H. Steele, ed., Encyclopedia of Ocean Sciences (Second Edition). second edition edn, Academic Press, Oxford, pp. 473–484. https://www.sciencedirect.com/science/article/pii/B978012374473900730X
Birkebak, M., Eren, F., Pe’eri, S. and Weston, N. (2018a). The Effect of Surface Waves on Airborne LiDAR Bathymetry (ALB) Measurement Uncertainties. Remote Sensing 10(3), 453. http://www.mdpi.com/2072-4292/10/3/453
Birkebak, M., Eren, F., Pe’eri, S. and Weston, N. (2018b). The effect of surface waves on airborne LiDAR bathymetry (ALB) measurement uncertainties. Remote Sensing 10(3).
Bleier, M., van der Lucht, J. and Nüchter, A. (2019). Scout3d – an underwater laser scanning system for mobile mapping. The International Archives of the Photogrammetry, Remote Sensing and Spatial Information Sci-ences XLII-2/W18, 13–18. https://www.int-arch-photogramm-remote-sens-spatial-inf-sci.net/XLII-2-W18/13/2019/
Botelho, S., Oliveira, G., Drews, P., Figueiredo, M. and Haffele, C. (2010). Visual odometry and mapping for underwater autonomous vehicles. H. Yussof, ed., Robot Localization and Map Buil-ding. IntechOpen, Rijeka, chapter 19. https://doi.org/10.5772/9274
Bundler (2022). Bundler – Structure from Motion for Unordered Image Collections. https://www.cs.cornell.edu/ snavely/bundler
Burns, J. H. R. and Delparte, D. (2017). Comparision of commercial Structure-from-Motion photogrammetry software used for underwater three-dimensional modeling of coral reef environments. The International Archives of the Photogrammetry, Remote Sensing and Spatial Information Sciences. Vol. XLII-2/W3, Nafplio, Greece, pp. 127–131.
Butler, J., Lane, S., Chandler, J. and Porfiri, E. (2002). Through-Water Close Range Digital Photogrammetry in Flume and Field Environments. The Photogrammetric Record 17(99), 419–439. https://onlinelibrary.wiley.com/doi/abs/10.1111/0031-868X.00196
Cahyono, A. B., Wibisono, A. C., Saptarini, D., Permadi, R. I., Budisusanto, Y. and Hidayat, H. (2020). Under-water photogrammetry application for coral reef mapping and monitoring. International Journal on Advanced Science, Engineering and Information Technology 10(1), 293–297. http://insightsociety.org/ojaseit/index.php/ijaseit/article/view/6747
Cao, B., Fang, Y., Gao, L., Hu, H., Jiang, Z., Sun, B. and Lou, L. (2021). An active-passive fusion strategy and accuracy evaluation for shallow water bathymetry based on ICESat-2 ATLAS laser point cloud and satellite remote sensing imagery. International Journal of Remote Sensing 42(8), pp. 2783–2806. https://doi.org/10.1080/01431161.2020.1862441
Cao, B., Fang, Y., Jiang, Z., Gao, L. and Hu, H. (2019). Shallow water bathymetry from WorldView-2 stereo imagery using two-media photogrammetry. European Journal of Remote Sensing 52(1), 506–521. https://doi.org/10.1080/22797254.2019.1658542
Capocci, R., Dooly, G., Omerdic, E., Coleman, J., Newe, T. and Toal, D. (2017). Inspection-class remotely operated vehicles—a review. Journal of Marine Science and Engineering 5(1). https://www.mdpi.com/2077-1312/5/1/13
Carrivick, J. L. and Smith, M. W. (2019). Fluvial and aquatic applications of Structure from Motion photogrammetry and unmanned aerial vehicle/drone technology. WIREs Water 6(1), e1328. https://onlinelibrary.wiley.com/doi/abs/10.1002/wat2.1328
Casella, E., Lewin, P., Ghilardi, M., Rovere, A. and Bejarano, S. (2022). Assessing the relative accuracy of coral heights reconstructed from drones and structure from motion photogrammetry on coral reefs. Coral Reefs 41(4), pp. 869–875. https://doi.org/10.1007/s00338-022-02244-9
Castillón, M., Palomer, A., Forest, J. and Ridao, P. (2019). State of the Art of Underwater Active Optical 3D Scanners. Sensors 19(23). https://www.mdpi.com/1424-8220/19/23/5161
Chang, A., Jung, J., Um, D., Yeom, J. and Hanselmann, F. (2019). Cost-effective framework for rapid underwater mapping with digital camera and color correction method. KSCE Journal of Civil Engineering 23(4), pp. 1776–1785. https://doi.org/10.1007/s12205-019-1891-3
Chemisky, B., Menna, F., Nocerino, E. and Drap, P. (2021). Underwater Survey for Oil and Gas Industry: A Review of Close Range Optical Methods. Remote Sensing 13(14). https://www.mdpi.com/2072-4292/13/14/2789
Christiansen, L. (2016). New techniques in capturing and modelling of morphological data. Hydrographische Nachrichten 105(11), pp. 22–25.
Christiansen, L. (2021). Laser bathymetry for coastal protection in schleswig-holstein. PFG – Journal of Photogrammetry, Remote Sensing and Geoinformation Science 89(2), 183–189. https://doi.org/10.1007/s41064-021-00149-w
Colomina, I. and Molina, P. (2014). Unmanned aerial systems for photogrammetry and remote sensing: A review. ISPRS Journal of Photogrammetry and Remote Sensing 92, pp. 79–97. http://www.sciencedirect.com/science/article/pii/S0924271614000501
Corcoran, F. and Parrish, C. E. (2021). Diffuse Attenuation Coefficient (Kd ) from ICESat-2 ATLAS Spaceborne LiDAR Using Random-Forest Regression. Photogrammetric Engineering and Remote Sensing 87(11), pp. 831–840. https://www.ingentaconnect.com/content/asprs/pers/2021/00000087/00000011/art00011
Costa, W. L. L., Bryan, K. R. and Coco, G. (2022). Modelling tides and storm surge using intertidal bathymetry derived from the waterline method applied to multispectral satellite images. Natural Hazards and Earth System Sciences Discussions 2022, pp. 1–24. https://nhess.copernicus.org/preprints/nhess-2021-387/
Daly, C., Baba, W., Bergsma, E., Thoumyre, G., Almar, R. and Garlan, T. (2022). The new era of regional coastal bathymetry from space: A showcase for west africa using optical sentinel-2 imagery. Remote Sensing of Environment 278, 113084. https://www.sciencedirect.com/science/article/pii/S0034425722001985
Degnan, J. J. (2016). Scanning, Multibeam, Single Photon Lidars for Rapid, Large Scale, High Resolution, Topographic and Bathymetric Mapping. Remote Sensing 8(11), pp. 923–958. http://www.mdpi.com/2072-4292/8/11/958
Dewi, R. S., Sofian, I. and Suprajaka (2022). The application of satellite derived bathymetry for coastline map-ping. IOP Conference Series: Earth and Environmental Science 950(1), 012088. https://doi.org/10.1088/1755-1315/950/1/012088
Dickens, K. and Armstrong, A. (2019). Application of Machine Learning in Satellite Derived Bathymetry and Coastline Detection. SMU Data Science Review 2(1).
Dietrich, J. T. (2016). Bathymetric Structure-from-Motion: extracting shallow stream bathymetry from multi-view stereo photogrammetry. Earth Surface Processes and Landforms 42(2), 355–364. https://onlinelibrary.wiley.com/doi/abs/10.1002/esp.4060
Doneus, M., Doneus, N., Briese, C., Pregesbauer, M., Mandlburger, G. and Verhoeven, G. (2013). Airborne laser bathymetry – detecting and recording submerged archaeological sites from the air. Journal of Archaeological Science 40(4).
Doneus, M., Miholjek, I., Mandlburger, G., Doneus, N., Verhoeven, G., Briese, C. and Pregesbauer, M. (2015). Airborne laser bathymetry for documentation of submerged archaeological sites in shallow water. International Archives of the Photogrammetry, Remote Sensing and Spatial Information Sciences – ISPRS Archives. Vol. 40.
Dramsch, J. S. (2020). 70 years of machine learning in geoscience in review. B. Moseley and L. Krischer, eds, Machine Learning in Geosciences. Vol. 61 of Advances in Geophysics, Elsevier, pp. 1–55. https://www.sciencedirect.com/science/article/pii/S0065268720300054
Drap, P. (2012). Underwater photogrammetry for archaeology. D. C. da Silva, ed., Special Applications of Photogrammetry. IntechOpen, Rijeka, chapter 6. https://doi.org/10.5772/33999
Duan, Z., Chu, S., Cheng, L., Ji, C., Li, M. and Shen, W. (2022). Satellite-derived bathymetry using Landsat-8 and Sentinel-2A images: assessment of atmospheric correction algorithms and depth derivation models in shallow waters. Opt. Express 30(3), 3238–3261. http://opg.optica.org/oe/abstract.cfm?URI=oe-30-3-3238
Effler, S. W. (1988). Secchi disc transparency and turbidity. Journal of Environmental Engineering 114(6), 1436–1447. https://ascelibrary.org/doi/abs/10.1061/%28ASCE%290733-9372%281988%29114%3A6%281436%29
Ellmer, W. (2016). Use of laser bathymetry at the German Baltic Sea coast. Hydrographische Nachrichten 105(11), 26–28.
Engelen, L., Creëlle, S., Schindfessel, L. and Mulder, T. D. (2018). Spatio-temporal image-based parametric water surface reconstruction: a novel methodology based on refraction. Measurement Science and Technology 29(3), 035302. https://doi.org/10.1088/1361-6501/aa9eb7
Eren, F., Jung, J., Parrish, C. E., Sarkozi-Forfinski, N. and Calder, B. R. (2019). Total Vertical Uncertainty (TVU) Modeling for Topo-Bathymetric LiDAR Systems. Photogrammetric Engineering and Remote Sensing 85(8), pp. 585–596. https://www.ingentaconnect.com/content/asprs/pers/2019/00000085/00000008/art00011
Escobar Villanueva, J. R., Iglesias Martínez, L. and Pérez Montiel, J. I. (2019). DEM Generation from Fixed-Wing UAV Imaging and LiDAR-Derived Ground Control Points for Flood Estimations. Sensors 19(14), 3205.
Eugenio, F., Marcello, J., Mederos-Barrera, A. and Marqués, F. (2022). High-resolution satellite bathymetry mapping: Regression and machine learning-based approaches. IEEE Transactions on Geoscience and Remote Sensing 60, pp. 1–14.
Ewing, G. C., ed. (1965), Oceanography from space, WHOI Ref. No. 65-10, Woods Hole Oceanographic Institution, Woods Hole, Massachusetts.
Fernandez-Diaz, J. C., Carter, W. E., Glennie, C., Shrestha, R. L., Pan, Z., Ekhtari, N., Singhania, A., Hauser, D. and Sartori, M. (2016). Capability Assessment and Performance Metrics for the Titan Multispectral Mapping LiDAR. Remote Sensing 8(11), 936. http://www.mdpi.com/2072-4292/8/11/936
Filisetti, A., Marouchos, A., Martini, A., Martin, T. and Collings, S. (2018). Developments and applications of underwater LiDAR systems in support of marine science. OCEANS 2018 MTS/IEEE Charleston. pp. 1–10.
Förstner, W. and Wrobel, B. P. (2016). Photogrammetric Computer Vision: Statistics, Geometry, Orientation and Reconstruction, Springer International Publishing, Cham, Switzerland, pp. 643–725. http://dx.doi.org/10.1007/978-3-319-11550-4 15
Fuchs, E. and Tuell, G. (2010). Conceptual design of the CZMIL data acquisition system (DAS): integrating a new bathymetric LiDAR with a commercial spectrometer and metric camera for coastal mapping applications. Algorithms and Technologies for Multispectral, Hyperspectral, and Ultraspectral Imagery XVI 7695(May 2010), 76950U.
Gasica, T. A. and Pratomo, D. G. (2022). Shallow waters depth estimation using empirical satellite derived bathymetry and Sentinel-2 data, case study: East coastal waters of Java island. The International Archives of the Photogrammetry, Remote Sensing and Spatial Information Sciences XLIII-B3-2022, pp. 93–99. https://www.int-arch-photogramm-remote-sens-spatial-inf-sci.net/XLIII-B3-2022/93/2022/
Gentile, V., Mróz, M., Spitoni, M., Lejot, J., Piégay, H. and Demarchi, L. (2016). Bathymetric Mapping of Shallow Rivers with UAV Hyperspectral Data. Proc. of the 5th International Conference on Telecommunications and Remote Sensing – Volume 1: ICTRS,. INSTICC, SciTePress, pp. 43–49.
Grobbelaar, J. (2009). Turbidity. G. E. Likens, ed., Encyclopedia of Inland Waters. Academic Press, Oxford, pp. 699–704. https://www.sciencedirect.com/science/article/pii/B9780123706263000752
Guenther, G., Cunningham, A., Laroque, P. and Reid, D. (2000). Meeting the accuracy challenge in airborne LiDAR bathymetry. Proceedings of the 20th EARSeL Symposium: Workshop on LiDAR Remote Sensing of Land and Sea. Dresden, Germany.
Guo, K., Li, Q., Wang, C., Mao, Q., Liu, Y., Zhu, J. and Wu, A. (2022). Development of a single-wavelength airborne bathymetric LiDAR: System design and data processing. ISPRS Journal of Photogrammetry and Remote Sensing 185, pp. 62–84. https://www.sciencedirect.com/science/article/pii/S0924271622000156
Haala, N. and Rothermel, M. (2012). Dense Multi-Stereo Matching for High Quality Digital Elevation Models. PFG Photogrammetrie, Fernerkundung, Geoinformation 2012(4), 331–343. http://www.ingentaconnect.com/content/schweiz/pfg/2012/00002012/00000004/art00003
Hartmann, K., Albada, E. and Heege, T. (2021). Latest developments in satellite derived bathymetry: Technology, use cases and tools. OCEANS 2021: San Diego – Porto. pp. 1–7.
Hatcher, G. A., Warrick, J. A., Ritchie, A. C., Dailey, E. T., Zawada, D. G., Kranenburg, C. and Yates, K. K. (2020). Accurate Bathymetric Maps From Underwater Digital Imagery Without Ground Control. Frontiers in Marine Science 7. https://www.frontiersin.org/articles/10.3389/fmars.2020.00525
He, J., Lin, J., Ma, M. and Liao, X. (2021). Mapping topo-bathymetry of transparent tufa lakes using UAV-based photogrammetry and RGB imagery. Geomorphology 389, 107832. https://www.sciencedirect.com/science/article/pii/S0169555X21002403
Herrmann, J., Magruder, L. A., Markel, J. and Parrish, C. E. (2022). Assessing the Ability to Quantify Bathymetric Change over Time Using Solely Satellite-Based Measurements. Remote Sensing 14(5). https://www.mdpi.com/2072-4292/14/5/1232
Hickman, G. D. and Hogg, J. E. (1969). Application of an airborne pulsed laser for near shore bathymetric measurements. Remote Sensing of Environment 1(1), pp. 47–58. http://www.sciencedirect.com/science/article/pii/S0034425769900881
Hirschmuller, H. (2008). Stereo Processing by Semiglobal Matching and Mutual Information. IEEE Trans. Pattern Anal. Mach. Intell. 30(2), 328–341. http://dx.doi.org/10.1109/TPAMI.2007.1166
Hodül, M., Bird, S., Knudby, A. and Chénier, R. (2018). Satellite derived photogrammetric bathymetry. ISPRS Journal of Photogrammetry and Remote Sensing 142, pp. 268–277. http://www.sciencedirect.com/science/article/pii/S0924271618301783
Horn, L. R., Uda, P. K., Franco, D. and Kern, P. (2022). Statistical analysis for determination of homogeneous reflectance regions in a coastal lagoon using satellite imagery and bathymetry survey. Journal of Applied Remote Sensing 16(3), 034508. https://doi.org/10.1117/1.JRS.16.034508
Hu, B., Zhao, Y., Chen, R., Liu, Q., Wang, P. and Zhang, Q. (2022). Denoising method for a LiDAR bathymetry system based on a low-rank recovery of non-local data structures. Appl. Opt. 61(1), 69–76. http://opg.optica.org/ao/abstract.cfm?URI=ao-61-1-69
IHO (2020). S-44, Standards for Hydrographic Surveys, Edition 6.0, Standard 5th ed., International Hydro-graphic Organization, Monaco. http://www.iho.int/iho pubs/standard/S-44 5E.pdf
IHO (2022). Official website of the International Hydrographic Organzation (IHO). https://iho.int/en/importance-of-hydrography
Islam, M. T., Yoshida, K., Nishiyama, S., Sakai, K., Adachi, S. and Pan, S. (2022). Promises and uncertainties in remotely sensed riverine hydro-environmental attributes: Field testing of novel approaches to unmanned aerial vehicle-borne LiDAR and imaging velocimetry. River Research and Applications n/a(n/a). https://onlinelibrary.wiley.com/doi/abs/10.1002/rra.4042
Janowski, L., Wroblewski, R., Rucinska, M., Kubowicz-Grajewska, A. and Tysiac, P. (2022). Automatic classifi-cation and mapping of the seabed using airborne LiDAR bathymetry. Engineering Geology 301, 106615. https://www.sciencedirect.com/science/article/pii/S0013795222001004
Ji, X., Yang, B., Tang, Q., Xu, W. and Li, J. (2022). Feature fusion-based registration of satellite images to airborne LiDAR bathymetry in island area. International Journal of Applied Earth Observation and Geoinformation 109, 102778. https://www.sciencedirect.com/science/article/pii/S0303243422001040
Ji, X., Yang, B., Wang, Y., Tang, Q. and Xu, W. (2022). Full-waveform classification and segmentation-based signal detection of single-wavelength bathymetric LiDAR. IEEE Transactions on Geoscience and Remote Sensing 60, pp. 1–14.
Jung, J., Lee, J. and Parrish, C. E. (2021). Inverse Histogram-Based Clustering Approach to Seafloor Segmentation from Bathymetric LiDAR Data. Remote Sensing 13(18). https://www.mdpi.com/2072-4292/13/18/3665
Kahmen, O., Rofallski, R. and Luhmann, T. (2020). Impact of stereo camera calibration to object accuracy in multimedia photogrammetry. Remote Sensing 12(12). https://www.mdpi.com/2072-4292/12/12/2057
Kay, S., Hedley, J. D. and Lavender, S. (2009). Sun glint correction of high and low spatial resolution images of aquatic scenes: a review of methods for visible and near-infrared wavelengths. Remote Sensing 1(4), 697–730. https://www.mdpi.com/2072-4292/1/4/697
Kobryn, H. T., Beckley, L. E. and Wouters, K. (2022). Bathymetry Derivatives and Habitat Data from Hyperspectral Imagery Establish a High-Resolution Baseline for Managing the Ningaloo Reef, Western Australia. Remote Sensing 14(8). https://www.mdpi.com/2072-4292/14/8/1827
Kogut, T. and Baku la, K. (2019). Improvement of Full Waveform Airborne Laser Bathymetry Data Processing based on Waves of Neighborhood Points. Remote Sensing 11(10). https://www.mdpi.com/2072-4292/11/10/1255
Kogut, T. and Slowik, A. (2021). Classification of Airborne Laser Bathymetry Data Using Artificial Neural Networks. IEEE Journal of Selected Topics in Applied Earth Observations and Remote Sensing 14, pp. 1959–1966.
Kogut, T., Tomczak, A., Slowik, A. and Oberski, T. (2022). Seabed modelling by means of airborne laservbathymetry data and imbalanced learning for offshore mapping. Sensors 22(9). https://www.mdpi.com/1424-8220/22/9/3121
Kotowski, R. (1988). Phototriangulation in Multi-Media-Photogrammetry. Int. Arch. Photogramm. Remote Sens. XXVII. Kyoto, pp. 324–334.
Koutalakis, P. and Zaimes, G. N. (2022). River Flow Measurements Utilizing UAV-Based Surface Velocimetry and Bathymetry Coupled with Sonar. Hydrology 9(8). https://www.mdpi.com/2306-5338/9/8/148
Kraus, K. (2007). Photogrammetry – Geometry from Images and Laser Scans, 2 edn, De Gruyter, Berlin, Germany.
Kreibich, H., Van Loon, A. F., Schröter, K. et al. (2022). The challenge of unprecedented floods and droughts in risk management. Nature 608(7921), pp. 80–86. https://doi.org/10.1038/s41586-022-04917-5
Kühne, E. (2021). New opportunities for capturing the topography of the river Elbe by airborne hydromapping in a low discharge period 2018. PFG – Journal of Photogrammetry, Remote Sensing and Geoinformation Science 89(2), pp. 177–182. https://doi.org/10.1007/s41064-021-00151-2
Kwasnitschka, T., Köser, K., Sticklus, J., Rothenbeck, M., Weiß, T., Wenzlaff, E., Schoening, T., Triebe, L., Steinführer, A., Devey, C. and Greinert, J. (2016). DeepSurveyCam—A Deep Ocean Optical Mapping System. Sensors 16(2). https://www.mdpi.com/1424-8220/16/2/164
Lague, D. and Feldmann, B. (2020). Topo-bathymetric airborne LiDAR for fluvial-geomorphology analysis. P. Tarolli and S. M. Mudd, eds, Remote Sensing of Geomorphology. Vol. 23 of Developments in Earth Surface Processes, Elsevier, pp. 25–54. https://www.sciencedirect.com/science/article/pii/B9780444641779000023
Lawen, J., Lawen, K., Salman, G. and Schuster, A. (2022). Multi-band bathymetry mapping with spiking neuron anomaly detection. Water 14(5). https://www.mdpi.com/2073-4441/14/5/810
Le Quilleuc, A., Collin, A., Jasinski, M. F. and Devillers, R. (2022). Very High-Resolution Satellite-Derived Bathymetry and Habitat Mapping Using Pleiades-1 and ICESat-2. Remote Sensing 14(1). https://www.mdpi.com/2072-4292/14/1/133
Le, Y., Hu, M., Chen, Y., Yan, Q., Zhang, D., Li, S., Zhang, X. and Wang, L. (2022). Investigating the Shallow-Water Bathymetric Capability of Zhuhai-1 Spaceborne Hyperspectral Images Based on ICESat-2 Data and Empirical Approaches: A Case Study in the South China Sea. Remote Sensing 14(14). https://www.mdpi.com/2072-4292/14/14/3406
Lee, J., Shin, H. and Lee, S. (2021). Development of a Wide Area 3D Scanning System with a Rotating Line Laser. Sensors 21(11). https://www.mdpi.com/1424-8220/21/11/3885
Legleiter, C. J. (2016). Inferring river bathymetry via Image to Depth Quantile Transformation (IDQT). Water Resources Research 52(5), 3722–3741. https://agupubs.onlinelibrary.wiley.com/doi/abs/10.1002/2016WR018730
Legleiter, C. J., Dar, A. R. and Rick, L. L. (2009). Spectrally based remote sensing of river bathymetry. Earth Surface Processes and Landforms 34(8), pp. 1039–1059. https://onlinelibrary.wiley.com/doi/abs/10.1002/esp.1787
Legleiter, C. J. and Harrison, L. R. (2019). Remote Sensing of River Bathymetry: Evaluating a Range of Sensors, Platforms, and Algorithms on the Upper Sacramento River, California, USA. Water Resources Research 55(3), 2142–2169. https://agupubs.onlinelibrary.wiley.com/doi/abs/10.1029/2018WR023586
Legleiter, C. J. and Hodges, S. W. (2022). Mapping Benthic Algae and Cyanobacteria in River Channels from Aerial Photographs and Satellite Images: A Proof-of-Concept Investigation on the Buffalo National River, AR, USA. Remote Sensing 14(4). https://www.mdpi.com/2072-4292/14/4/953
Letard, M., Collin, A., Corpetti, T., Lague, D., Pastol, Y. and Ekelund, A. (2022). Classification of land-water continuum habitats using exclusively airborne topobathymetric LiDAR green waveforms and infrared intensity point clouds. Remote Sensing 14(2). https://www.mdpi.com/2072-4292/14/2/341
Letard, M., Collin, A., Lague, D., Corpetti, T., Pastol, Y., Ekelund, A., Pergent, G. and Costa, S. (2021). Towards 3D mapping of seagrass meadows with topo-bathymetric LiDAR full waveform processing. IEEE IGARSS 2021. Brussels (virtual conference), Belgium. https://hal.archives-ouvertes.fr/hal-03279007
Li, J., Tao, B., He, Y., Li, Y., Huang, H., Mao, Z. and Yu, J. (2022). Range difference between shallow and deep channels of airborne bathymetry LiDAR with segmented field-of-view receivers. IEEE Transactions on Geoscience and Remote Sensing 60, pp. 1–16.
Li, S., Su, D., Yang, F., Zhang, H., Wang, X. and Guo, Y. (2022). Bathymetric LiDAR and multibeam echo-sounding data registration methodology employing a point cloud model. Applied Ocean Research 123, 103147. https://www.sciencedirect.com/science/article/pii/S0141118722000967
Liu, S., Wang, L., Liu, H., Su, H., Li, X. and Zheng, W. (2018). Deriving Bathymetry From Optical Images With a Localized Neural Network Algorithm. IEEE Transactions on Geoscience and Remote Sensing 56(9), pp. 5334–5342.
Lowell, K. and Calder, B. (2022). Operational performance of a combined density- and clustering-based approach to extract bathymetry returns from LiDAR point clouds. International Journal of Applied Earth Observation and Geoinformation 107, 102699. https://www.sciencedirect.com/science/article/pii/S0303243422000253
Lubczonek, J., Kazimierski, W., Zaniewicz, G. and Lacka, M. (2022). Methodology for combining data acquired by unmanned surface and aerial vehicles to create digital bathymetric models in shallow and ultra-shallow waters. Remote Sensing 14(1). https://www.mdpi.com/2072-4292/14/1/105
Luhmann, T., Robson, S., Kyle, S. and Boehm, J. (2019). Close-Range Photogrammetry and 3D Imaging, De Gruyter, Berlin, Boston. https://doi.org/10.1515/9783110607253
Lumban-Gaol, Y., Ohori, K. A. and Peters, R. (2022). Extracting Coastal Water Depths from Multi-Temporal Sentinel-2 Images Using Convolutional Neural Networks. Marine Geodesy 0(0), 1–30. https://doi.org/10.1080/01490419.2022.2091696
Lurton, X. (2010). An Introduction to Underwater Acoustics – Principles and Applications, 2 edn, Springer-Verlag Berlin Heidelberg, Berlin, Heidelberg.
Lyzenga, D. R. (1978). Passive remote sensing techniques for mapping water depth and bottom features. Applied Optics 17(3), pp. 379–383.
Lyzenga, D. R., Malinas, N. P. and Tanis, F. J. (2006). Multispectral bathymetry using a simple physically based algorithm. IEEE Transactions on Geoscience and Remote Sensing 44(8), 2251–2259.
Ma, T., Li, Y., Zhao, Y., Zhang, Q., Jiang, Y., Cong, Z. and Zhang, T. (2020). Robust bathymetric SLAM algorithm considering invalid loop closures. Applied Ocean Research 102, 102298. https://www.sciencedirect.com/science/article/pii/S0141118719308715
Maas, H.-G. (2015). On the Accuracy Potential in Underwater/Multimedia Photogrammetry. Sensors 15(8), 18140–18152. http://www.mdpi.com/1424-8220/15/8/18140
Macon, C. L. (2009). Usace national coastal mapping program and the next generation of data products. OCEANS 2009. pp. 1–7.
Mader, D., Richter, K., Westfeld, P. and Maas, H.-G. (2022). Potential of a Non-linear Full-Waveform Stacking Technique in Airborne LiDAR Bathymetry, PFG – Journal of Photogrammetry, Remote Sensing and Geoinformation Science. https://doi.org/10.1007/s41064-022-00212-0
Makboul, O., Negm, A., Mesbah, S. and Mohasseb, M. (2017). Performance Assessment of ANN in Estimating Remotely Sensed Extracted Bathymetry. Case Study: Eastern Harbor of Alexandria. Procedia Engineering 181, 912 – 919. 10th International Conference Interdisciplinarity in Engineering, INTER-ENG 2016, 6-7 October 2016, Tirgu Mures, Romania. http://www.sciencedirect.com/science/article/pii/S1877705817310767
Mandlburger, G. (2019). Through-water dense image matching for shallow water bathymetry. Photogrammetric Engineering and Remote Sensing 85(6).
Mandlburger, G. (2020). A review of airborne laser bathymetry for mapping of inland and coastal waters. Journal of Applied Hydrography 116, pp. 6–15.
Mandlburger, G., Hauer, C., Wieser, M. and Pfeifer, N. (2015). Topo-bathymetric LiDAR for monitoring river morphodynamics and instream habitats-A case study at the Pielach River. Remote Sensing 7(5), 6160–6195. http://www.mdpi.com/2072-4292/7/5/6160
Mandlburger, G. and Jutzi, B. (2019). On the feasibility of water surface mapping with single photon LiDAR. ISPRS International Journal of Geo-Information 8(4).
Mandlburger, G., Kölle, M., Nübel, H. and Sörgel, U. (2021). BathyNet: A Deep Neural Network for Water Depth Mapping from Multispectral Aerial Images. PFG – Journal of Photogrammetry, Remote Sensing and Geoinformation Science 89(2), pp. 71–89.
Mandlburger, G., Pfennigbauer, M. and Pfeifer, N. (2013). Analyzing near water surface penetration in laser bathymetry – A case study at the River Pielach. ISPRS Annals of the Photogrammetry, Remote Sensing and Spatial Information Sciences. Vol. II-5/W2, pp. 175–180. http://publik.tuwien.ac.at/files/PubDat 221149.pdf
Mandlburger, G., Pfennigbauer, M., Schwarz, R., Flöry, S. and Nussbaumer, L. (2020). Concept and Performance Evaluation of a Novel UAV-Borne Topo-Bathymetric LiDAR Sensor. Remote Sensing 12(6), 986. https://www.mdpi.com/2072-4292/12/6/986
Maritorena, S., Morel, A. and Gentili, B. (1994). Diffuse reflectance of oceanic shallow waters: Influence of water depth and bottom albedo. Limnology and Oceanography 39(7), 1689–1703. https://aslopubs.onlinelibrary.wiley.com/doi/abs/10.4319/lo.1994.39.7.1689
Markus, T., Neumann, T., Martino, A., et al. (2017). The Ice, Cloud, and land Elevation Satellite-2 (ICESat-2): Science requirements, concept, and implementation. Remote Sensing of Environment 190, 260–273. https://www.sciencedirect.com/science/article/pii/S0034425716305089
Massot-Campos, M. and Oliver-Codina, G. (2015). Optical Sensors and Methods for Underwater 3D Reconstruction. Sensors 15(12), 31525–31557. https://www.mdpi.com/1424-8220/15/12/29864
Massot-Campos, M., Oliver, G., Bodenmann, A. and Thornton, B. (2016). Submap bathymetric SLAM using structured light in underwater environments. 2016 IEEE/OES Autonomous Underwater Vehicles (AUV). pp. 181–188.
McKean, J., Isaak, D. and Wright, W. (2009). Improving Stream Studies With a Small-Footprint Green LiDAR. Eos, Transactions American Geophysical Union 90(39), 341–342. https://agupubs.onlinelibrary.wiley.com/doi/abs/10.1029/2009EO390002
McKean, J., Nagel, D., Tonina, D., Bailey, P., Wright, C. W., Bohn, C. and Nayegandhi, A. (2009). Remote Sensing of Channels and Riparian Zones with a Narrow-Beam Aquatic-Terrestrial LiDAR. Remote Sensing 1(4), 1065–1096. https://www.mdpi.com/2072-4292/1/4/1065
Menna, F., Nocerino, E., Fassi, F. and Remondino, F. (2016). Geometric and optic characterization of a hemi-spherical dome port for underwater photogrammetry. Sensors (Switzerland) 16(1), pp. 1–21.
Menna, F., Nocerino, E., Malek, S., Remondino, F. and Schiaparelli, S. (2022). A combined approach for long-term monitoring of benthos in antarctica with underwater photogrammetry and image understanding. The International Archives of the Photogrammetry, Remote Sensing and Spatial Information Sciences XLIII-B2-2022, 935–943. https://www.int-arch-photogramm-remote-sens-spatial-inf-sci.net/XLIII-B2-2022/935/2022/
Menna, F., Nocerino, E. and Remondino, F. (2017a). Flat versus hemispherical dome ports in underwater photogrammetry. The International Archives of the Photogrammetry, Remote Sensing and Spatial Information Sciences XLII-2/W3, 481–487. https://www.int-arch-photogramm-remote-sens-spatial-inf-sci.net/XLII-2-W3/481/2017/
Menna, F., Nocerino, E. and Remondino, F. (2017b). Optical aberrations in underwater photogrammetry with flat and hemispherical dome ports. F. Remondino and M. R. Shortis, eds, Videometrics, Range Imaging, and Applications XIV. International Society for Optics and Photonics, SPIE, p. 1033205. https://doi.org/10.1117/12.2270765
Misra, A., Vojinovic, Z., Ramakrishnan, B., Luijendijk, A. and Ranasinghe, R. (2018). Shallow water bathymetry mapping using Support Vector Machine (SVM) technique and multispectral imagery. International Journal of Remote Sensing 39(13), pp. 4431–4450. https://doi.org/10.1080/01431161.2017.1421796
Mitchell, S. E. and Thayer, J. P. (2014). Ranging through shallow semitransparent media with polarization LiDAR. Journal of Atmospheric and Oceanic Technology 31(3), 681 – 697. https://journals.ametsoc.org/view/journals/atot/31/3/jtech-d-13-00014 1.xml
Mitchell, T. (2019). From PILLS To RAMMS. 20th Annual JALBTCX Airborne Coastal Mapping and Charting Technical Workshop.
Moran, N., Stringer, B., Lin, B. and Hoque, M. T. (2022). Machine learning model selection for predicting bathymetry. Deep Sea Research Part I: Oceanographic Research Papers 185, 103788. https://www.sciencedirect.com/science/article/pii/S0967063722001017
Mudiyanselage, S., Abd-Elrahman, A., Wilkinson, B. and Lecours, V. (2022). Satellite-derived bathymetry using machine learning and optimal Sentinel-2 imagery in South-West Florida coastal waters. GIScience and Remote Sensing 59(1), pp. 1143–1158. https://doi.org/10.1080/15481603.2022.2100597
Mulsow, C. (2010). A flexible multi-media bundle approach. The International Archives of the Photogramme-try, Remote Sensing and Spatial Information Sciences. Vol. XXXVIII-5, Newcastle upon Tyne, pp. 472–477.
Murase, T., Tanaka, M., Tani, T., Miyashita, Y., Ohkawa, N., Ishiguro, S., Suzuki, Y., Kayanne, H. and Yamano, H. (2008). A photogrammetric correction procedure for light refraction effects at a two-medium boundary. Photogrammetric Engineering and Remote Sensing 74, 1129–1136.
Murtagh, F. (1991). Multilayer perceptrons for classification and regression. Neurocomputing 2(5), 183–197. https://www.sciencedirect.com/science/article/pii/0925231291900235
Najar, M. A., Benshila, R., Bennioui, Y. E., Thoumyre, G., Almar, R., Bergsma, E. W. J., Delvit, J.-M. and Wilson, D. G. (2022). Coastal Bathymetry Estimation from Sentinel-2 Satellite Imagery: Comparing Deep Learning and Physics-Based Approaches. Remote Sensing 14(5). https://www.mdpi.com/2072-4292/14/5/1196
Neumann, T. A., Martino, A. J., Markus, T., et al. (2019). The Ice, Cloud, and Land Elevation Satellite – 2 mission: A global geolocated photon product derived from the Advanced Topographic Laser Altimeter System. Remote Sensing of Environment 233, 111325. https://www.sciencedirect.com/science/article/pii/S003442571930344X
nFrames (2022). SURE aerial – Software for Photomodeller – Software fro photogrammetric measurements and mapping from photos. https://www.nframes.com/products/sure-aerial
Niroumand-Jadidi, M., Legleiter, C. J. and Bovolo, F. (2022a). Bathymetry retrieval from cubesat image sequences with short time lags. International Journal of Applied Earth Observation and Geoinformation 112, 102958. https://www.sciencedirect.com/science/article/pii/S1569843222001534
Niroumand-Jadidi, M., Legleiter, C. J. and Bovolo, F. (2022b). River Bathymetry Retrieval From Landsat-9 Images Based on Neural Networks and Comparison to SuperDove and Sentinel-2. IEEE Journal of Selected Topics in Applied Earth Observations and Remote Sensing 15, pp. 5250–5260.
NOAA (2022). Coastal Topobathy LiDAR. https://coast.noaa.gov/digitalcoast/data/jalbtcx.html
Nocerino, E. and Menna, F. (2020). Photogrammetry: Linking the world across the water surface. Journal of Marine Science and Engineering 8(2). https://www.mdpi.com/2077-1312/8/2/128
Nocerino, E., Menna, F., Gruen, A., Troyer, M., Capra, A., Castagnetti, C., Rossi, P., Brooks, A. J., Schmitt, R. J. and Holbrook, S. J. (2020). Coral reef monitoring by scuba divers using underwater photogrammetry and geodetic surveying. Remote Sensing 12(18). https://www.mdpi.com/2072-4292/12/18/3036
Parrish, C. E., Dijkstra, J. A., O’Neil-Dunne, J. P. M., McKenna, L. and Pe’eri, S. (2016). Post-Sandy Benthic Habitat Mapping Using New Topobathymetric LiDAR Technology and Object-Based Image Classification. Journal of Coastal Research pp. 200–208. https://doi.org/10.2112/SI76-017
Parrish, C. E., Magruder, L. A., Neuenschwander, A. L., Forfinski-Sarkozi, N., Alonzo, M. and Jasinski, M. (2019). Validation of ICESat-2 ATLAS bathymetry and analysis of ATLAS’s bathymetric mapping performance. Remote Sensing 11(14).
Pfennigbauer, M., Rieger, P., Schwarz, R. and Ullrich, A. (2022). Impact of beam parameters on the performance of a topo-bathymetric LiDAR sensor. G. W. Kamerman, L. A. Magruder and M. D. Turner, eds, Laser Radar Technology and Applications XXVII. Vol. 12110, International Society for Optics and Photonics, SPIE, p. 121100C. https://doi.org/10.1117/12.2618794
Pfennigbauer, M. and Ullrich, A. (2010). Improving quality of laser scanning data acquisition through calibrated amplitude and pulse deviation measurement. Proc. SPIE. Vol. 7684, pp. 7684ff. https://doi.org/10.1117/12.849641
Pfennigbauer, M., Ullrich, A., Steinbacher, F. and Aufleger, M. (2011). High-resolution hydrographic airborne laser scanner for surveying inland waters and shallow coastal zones. Proc. SPIE. Vol. 8037, pp. 803706–803711. http://dx.doi.org/10.1117/12.883910
Pfennigbauer, M., Wolf, C., Weinkopf, J. and Ullrich, A. (2014). Online waveform processing for demanding target situations. Proc. SPIE. p. 90800J. http://proceedings.spiedigitallibrary.org/proceeding.aspx?doi=10.1117/12.2052994
Philpot, W. D. (1989). Bathymetric mapping with passive multispectral imagery. Appl. Opt. 28(8), pp. 1569–1578. http://opg.optica.org/ao/abstract.cfm?URI=ao-28-8-1569
Philpot, W., ed. (2019). Airborne Laser Hydrography II, Cornell University Library (eCommons), Coernell. https://ecommons.cornell.edu/handle/1813/66666
Piazza, P., Cummings, V., Lohrer, D., Marini, S., Marriott, P., Menna, F., Nocerino, E., Peirano, A. and Schiaparelli, S. (2018). Divers-operated underwater photogrammetry: Applications in the study of antarctic benthos. The International Archives of the Photogrammetry, Remote Sensing and Spatial Information Sciences XLII-2, pp. 885–892. https://www.int-arch-photogramm-remote-sens-spatial-inf-sci.net/XLII-2/885/2018/
Pix4D (2022). Pix4Dmapper: professional drone mapping and photogrammetry software. https://www.pix4d.com/product/pix4dmapper-photogrammetry-software
Poicyn, F. C., Brown, W. and Sattinger, I. J. (1970). The measurement of water depth by remote sensing techniques, Technical Report 8973-26-F, Willow Run Laboratory, The University of Michigan.
Pope, R. M. and Fry, E. S. (1997). Absorption spectrum (380–700 nm) of pure water. II. Integrating cavity measurements. Appl. Opt. 36(33), pp. 8710–8723. http://opg.optica.org/ao/abstract.cfm?URI=ao-36-33-8710
Quadros, N. D. (2013). Unlocking the characteristics of bathymetric LiDAR sensors. http://www.lidarmag.com/PDF/LiDARMagazine Quadros-BathymetricLiDARSensors Vol3No6.pdf
Richter, K., Maas, H.-G., Westfeld, P. and Weiß, R. (2017). An Approach to Determining Turbidity and Correcting for Signal Attenuation in Airborne LiDAR Bathymetry. PFG – Journal of Photogrammetry, Remote Sensing and Geoinformation Science 85(1), pp. 31–40. https://doi.org/10.1007/s41064-016-0001-0
Richter, K., Mader, D., Westfeld, P. and Maas, H.-G. (2021a). Refined Geometric Modeling of Laser Pulse Propagation in Airborne LiDAR Bathymetry. PFG – Journal of Photogrammetry, Remote Sensing and Geoinformation Science 89(2), pp. 121–137. https://doi.org/10.1007/s41064-021-00146-z
Richter, K., Mader, D., Westfeld, P. and Maas, H.-G. (2021b). Water turbidity estimation from LiDAR bathymetry data by full-waveform analysis – comparison of two approaches. The International Archives of the Photogrammetry, Remote Sensing and Spatial Information Sciences XLIII-B2-2021, 681–688. https://www.int-arch-photogramm-remote-sens-spatial-inf-sci.net/XLIII-B2-2021/681/2021/
Rinner, K. (1948). Abbildungsgesetz und Orientierungsaufgaben in der Zweimedienphotogrammetrie. Österreichische Zeitschrift für Vermessungswesen Sonderheft(5).
Rofallski, R. and Luhmann, T. (2022). An efficient solution to ray tracing problems in multimedia photogrammetry for flat refractive interfaces. PFG – Journal of Photogrammetry, Remote Sensing and Geoinformation Science 90(1), 37–54. https://doi.org/10.1007/s41064-022-00192-1
Roshandel, S., Liu, W., Wang, C. and Li, J. (2021). 3D Ocean Water Wave Surface Analysis on Airborne LiDAR Bathymetric Point Clouds. Remote Sensing 13(19). https://www.mdpi.com/2072-4292/13/19/3918
Roshandel, S., Liu, W., Wang, C. and Li, J. (2022). Semantic segmentation of coastal zone on airborne LiDAR bathymetry point clouds. IEEE Geoscience and Remote Sensing Letters 19, pp. 1–5.
Rossi, L., Mammi, I. and Pelliccia, F. (2020). UAV-derived multispectral bathymetry. Remote Sensing 12(23). https://www.mdpi.com/2072-4292/12/23/3897
Rothermel, M., Wenzel, K., Fritsch, D. and Haala, N. (2012). SURE: Photogrammetric surface reconstruction from imagery. Proceedings of the Low Cost 3D Workshop, Berlin. Berlin. http://www.ifp.uni-stuttgart.de/publications/2012/Rothermel etal lc3d.pdf
Röttgers, R., McKee, D. and Utschig, C. (2014). Temperature and salinity correction coefficients for light absorption by water in the visible to infrared spectral region. Opt. Express 22(21), 25093–25108. http://opg.optica.org/oe/abstract.cfm?URI=oe-22-21-25093
Rupnik, E., Daakir, M. and Pierrot Deseilligny, M. (2017). MicMac – a free, open-source solution for photogrammetry. Open Geospatial Data, Software and Standards 14, 9. https://doi.org/10.1186/s40965-017-0027-2
Sagawa, T., Yamashita, Y., Okumura, T. and Yamanokuchi, T. (2019). Satellite Derived Bathymetry Using Machine Learning and Multi-Temporal Satellite Images. Remote Sensing 11(10). https://www.mdpi.com/2072-4292/11/10/1155
Sahoo, A., Dwivedy, S. K. and Robi, P. (2019). Advancements in the field of autonomous underwater vehicle. Ocean Engineering 181, 145–160. https://www.sciencedirect.com/science/article/pii/S0029801819301623
Salavitabar, S. and Li, S. S. (2022). Estimates of river bathymetry from satellite images: A case study of the nicolect river in quebec. S. Walbridge, M. Nik-Bakht, K. T. W. Ng, M. Shome, M. S. Alam, A. El Damatty and G. Lovegrove, eds, Proceedings of the Canadian Society of Civil Engineering Annual Conference 2021. Springer Nature Singapore, Singapore, pp. 401–411.
Salavitabar, S., Li, S. S. and Lak, B. (2022). Mapping underwater bathymetry of a shallow river from satellite multispectral imagery. Geosciences 12(4). https://www.mdpi.com/2076-3263/12/4/142
Sandwell, D. T., Müller, R. D., Smith, W. H. F., Garcia, E. and Francis, R. (2014). New global marine gravity model from Cryosat-2 and Jason-1 reveals buried tectonic structure. Science 346(6205), 65–67. https://science.sciencemag.org/content/346/6205/65
Santos, D., Fernández-Fernández, S., Abreu, T., Silva, P. A. and Baptista, P. (2022). Retrieval of nearshore bathymetry from Sentinel-1 SAR data in high energetic wave coasts: The Portuguese case study. Remote Sensing Applications: Society and Environment 25, 100674. https://www.sciencedirect.com/science/article/pii/S235293852100210X
Sardemann, H., Mulsow, C. and Maas, H.-G. (2022). Accuracy Analysis of an Oblique Underwater Laser Light-sheet Triangulation System. PFG – Journal of Photogrammetry, Remote Sensing and Geoinformation Science 90(1), pp. 3–18. https://doi.org/10.1007/s41064-022-00196-x
Saylam, K., Hupp, J. R., Averett, A. R., Gutelius, W. F. and Gelhar, B. W. (2018). Airborne LiDAR bathymetry: assessing quality assurance and quality control methods with Leica Chiroptera examples. International Journal of Remote Sensing 39(8), pp. 2518–2542. https://doi.org/10.1080/01431161.2018.1430916
Schonberger, J. L. and Frahm, J.-M. (2016). Structure-from-motion revisited. Proceedings of the IEEE Conference on Computer Vision and Pattern Recognition (CVPR).
Schwarz, R. K., Pfeifer, N., Pfennigbauer, M. and Mandlburger, G. (2021). Depth Measurement Bias in Pulsed Airborne Laser Hydrography Induced by Chromatic Dispersion. IEEE Geoscience and Remote Sensing Let-ters 18(8), pp. 1332–1336.
Schwarz, R., Mandlburger, G., Pfennigbauer, M. and Pfeifer, N. (2019). Design and evaluation of a full-wave surface and bottom-detection algorithm for LiDAR bathymetry of very shallow waters. ISPRS Journal of Photogrammetry and Remote Sensing 150.
Schwarz, R., Pfeifer, N., Pfennigbauer, M. and Ullrich, A. (2017). Exponential Decomposition with Implicit Deconvolution of LiDAR Backscatter from the Water Column. PFG – Journal of Photogrammetry, Remote Sensing and Geoinformation Science 85(3), pp. 159–167. https://doi.org/10.1007/s41064-017-0018-z
Shanmuga Priyaa, S., Aruna Kumar, A. and Jena, B. K. (2022). Bathymetry Retrieval Using Remote Sensing Techniques for Inter-tidal Regions of Tapi Estuary, Springer International Publishing, Cham, pp. 213–226. https://doi.org/10.1007/978-3-031-05057-2 19
Shen, X., Kong, W., Chen, P., Chen, T., Huang, G. and Shu, R. (2022). A shipborne photon-counting LiDAR for depth-resolved ocean observation. Remote Sensing 14(14). https://www.mdpi.com/2072-4292/14/14/3351
Shintani, C. and Fonstad, M. A. (2017). Comparing remote-sensing techniques collecting bathymetric data from a gravel-bed river. International Journal of Remote Sensing 38(8-10), 2883–2902. https://doi.org/10.1080/01431161.2017.1280636
Slocum, R. K., Parrish, C. E. and Simpson, C. H. (2020). Combined geometric-radiometric and neural network approach to shallow bathymetric mapping with UAS imagery. ISPRS Journal of Photogrammetry and Remote Sensing 169, pp. 351 – 363. http://www.sciencedirect.com/science/article/pii/S0924271620302434
Song, Y., Niemeyer, J., Ellmer, W., Soergel, U. and Heipke, C. (2015). Comparison of three airborne laser bathymetry data sets for monitoring the German Baltic Sea Coast. Proc. SPIE. Vol. 9638, pp. 9638 – 9638 – 9. https://doi.org/10.1117/12.2194960
Sonogashira, M., Shonai, M. and Iiyama, M. (2020). High-resolution bathymetry by deep-learning-based image superresolution. PLOS ONE 15(7), pp. 1–19. https://doi.org/10.1371/journal.pone.0235487
Sorenson, G., Honey, R. and Payne, J. (1966). Analysis of the use of airborne laser radar for submarine detection and ranging, Technical report, SRI Report No. 5583, Stanford Research Institute.
Specht, M., Wisniewska, M., Stateczny, A., Specht, C., Szostak, B., Lewicka, O., Stateczny, M., Widzgowski, S. and Halicki, A. (2022). Analysis of methods for determining shallow waterbody depths based on images taken by unmanned aerial vehicles. Sensors 22(5). https://www.mdpi.com/1424-8220/22/5/1844
Starek, M. J. and Giessel, J. (2017). Fusion of UAS-based structure-from-motion and optical inversion for seam-less topo-bathymetric mapping. 2017 IEEE International Geoscience and Remote Sensing Symposium (IGARSS). pp. 2999–3002.
Steinbacher, F., Dobler, W., Benger, W., Baran, R., Niederwieser, M. and Leimer, W. (2021). Integrated Full-Waveform Analysis and Classification Approaches for Topo-Bathymetric Data Processing and Visualization in HydroVISH. PFG – Journal of Photogrammetry, Remote Sensing and Geoinformation Science 89(2), pp. 159–175. https://doi.org/10.1007/s41064-021-00150-3
Stumpf, R. P., Holderied, K. and Sinclair, M. (2003). Determination of water depth with high-resolution satellite imagery over variable bottom types. Limnology and Oceanography 48(1part2), 547–556. https://aslopubs.onlinelibrary.wiley.com/doi/abs/10.4319/lo.2003.48.1 part 2.0547
Stumpf, R. P. and Pennock, J. R. (1989). Calibration of a general optical equation for remote sensing of suspended sediments in a moderately turbid estuary. Journal of Geophysical Research: Oceans 94(C10), pp. 14363–14371. https://agupubs.onlinelibrary.wiley.com/doi/abs/10.1029/JC094iC10p14363
Su, L. and Gibeaut, J. (2013). Mapping seagrass meadows in redfish bay with WorldView-2 imagery. American Society for Photogrammetry and Remote Sensing Annual Conference, ASPRS 2013.
Susa, T. (2022). Satellite derived bathymetry with Sentinel-2 imagery: Comparing traditional techniques with advanced methods and machine learning ensemble models. Marine Geodesy 45(5), 435–461. htps://doi.org/10.1080/01490419.2022.2064572
Templin, T., Popielarczyk, D. and Kosecki, R. (2018). Application of Low-Cost Fixed-Wing UAV for Inland Lakes Shoreline Investigation. Pure and Applied Geophysics 175(9), 3263–3283. https://doi.org/10.1007/s00024-017-1707-7
Thomas, N., Lee, B., Coutts, O., Bunting, P., Lagomasino, D. and Fatoyinbo, L. (2022). A purely spaceborne open source approach for regional bathymetry mapping. IEEE Transactions on Geoscience and Remote Sensing 60, pp. 1–9.
Thomas, R. and Guenther, G. (1990). Water surface detection strategy for an airborne laser bathymeter. Proc. SPIE. Vol. 1302, pp. 1302–1315. https://doi.org/10.1117/12.21474
Toschi, I., Remondino, F., Rothe, R. and Klimek, K. (2018). Combining Airborne Oblique Camera and LiDAR Sensors: Investigation and New Perspectives. International Archives of the Photogrammetry, Remote Sensing and Spatial Information Sciences. Vol. XLII, Karlsruhe, pp. 437–444.
Trimble (2022a). Inpho UASMaster – Complete Photogrammetric Workstation for UAS and Terrestrial Close-Range Imagery. https://geospatial.trimble.com/products-and-solutions/trimble-inpho-uasmaster
Trimble (2022b). Photomodeller – Software for city and countrywide mapping with aerial imagery. https://www.photomodeler.com
Trimble (2022c). RealityCapture – Photogrammetry software application for Windows. https://https://www.capturingreality.com/realitycapture
Tulldahl, H. M. and Steinvall, K. O. (2004). Simulation of sea surface wave influence on small target detection with airborne laser depth sounding. Appl. Opt. 43(12), pp. 2462–2483. http://ao.osa.org/abstract.cfm?URI=ao-43-12-2462
Ullrich, A. and Pfennigbauer, M. (2012). Laser hydrography. Patent US 8,307,705 B2. https://patentimages.storage.googleapis.com/a6/98/e3/ce103e00d84c4c/US8307705.pdf
Ullrich, A. and Pfennigbauer, M. (2016). Linear LiDAR versus Geiger-mode LiDAR: impact on data properties and data quality. Proc. SPIE. Vol. 9832, pp. 983204–983217. http://dx.doi.org/10.1117/12.2223586
Ventura, D. (2020). Coastal zone mapping with the world’s first airborne multibeam bathymetric LiDAR mapping system. Hydrographische Nachrichten. Vol. 115, Deutsche Hydrographische Gesellschaft e.V., pp. 48–53.
Wagner, W. (2010). Radiometric calibration of small-footprint full-waveform airborne laser scanner measurements: Basic physical concepts. ISPRS Journal of Photogrammetry and Remote Sensing 65(6), 505–513. http://dx.doi.org/10.1016/j.isprsjprs.2010.06.007
Wagner, W., Ullrich, A., Ducic, V., Melzer, T. and Studnicka, N. (2006). Gaussian decomposition and calibration of a novel small-footprint full-waveform digitising airborne laser scanner. ISPRS Journal of Photogrammetry and Remote Sensing 60(2), pp. 100–112.
Wang, C., Li, Q., Liu, Y., Wu, G., Liu, P. and Ding, X. (2015). A comparison of waveform processing algorithms for single-wavelength LiDAR bathymetry. ISPRS Journal of Photogrammetry and Remote Sensing 101, pp. 22–35. https://www.sciencedirect.com/science/article/pii/S0924271614002718
Wang, D., Xing, S., He, Y., Yu, J., Xu, Q. and Li, P. (2022). Evaluation of a New Lightweight UAV-Borne Topo-Bathymetric LiDAR for Shallow Water Bathymetry and Object Detection. Sensors 22(4). https://www.mdpi.com/1424-8220/22/4/1379
Wang, J., Chen, M., Zhu, W., Hu, L. and Wang, Y. (2022). A Combined Approach for Retrieving Bathymetry from Aerial Stereo RGB Imagery. Remote Sensing 14(3). https://www.mdpi.com/2072-4292/14/3/760
Watanabe, Y. and Kawahara, Y. (2016). UAV Photogrammetry for Monitoring Changes in River Topography and Vegetation. Procedia Engineering 154, pp. 317–325. http://www.sciencedirect.com/science/article/pii/S1877705816318719
Wedding, L. M., Friedlander, A. M., McGranaghan, M., Yost, R. S. and Monaco, M. E. (2008). Using bathymetric LiDAR to define nearshore benthic habitat complexity: Implications for management of reef fish assemblages in Hawaii. Remote Sensing of Environment 112(11), pp. 4159–4165.
Wenzel, K., Rothermel, M., Haala, N. and Fritsch, D. (2013). SURE – The ifp Software for Dense Image Matching. D. Fritsch, ed., Photogrammetric Week 13. Wichmann/VDE Verlag, Berlin and Offenbach, pp. 59–70. http://www.ifp.uni-stuttgart.de/publications/phowo13/080Wenzel.pdf
Westaway, R. M., Lane, S. N. and Hicks, D. M. (2001). Remote sensing of clear-water, shallow, gravel-bed rivers using digital photogrammetry. Photogrammetric Engineering and Remote Sensing 67(11), pp. 1271–1281.
Westfeld, P., Maas, H.-G., Richter, K. and Weiß, R. (2017). Analysis and correction of ocean wave pattern induced systematic coordinate errors in airborne LiDAR bathymetry. ISPRS Journal of Photogrammetry and Remote Sensing 128, pp. 314–325. http://dx.doi.org/10.1016/j.isprsjprs.2017.04.008
Wilson, N., Parrish, C. E., Battista, T., Wright, C. W., Costa, B., Slocum, R. K., Dijkstra, J. A. and Tyler, M. T. (2019). Mapping Seafloor Relative Reflectance and Assessing Coral Reef Morphology with EAARL-B Topobathymetric LiDAR Waveforms. Estuaries and Coasts .
Wozencraft, J. M. and Lillycrop, W. J. (2003). SHOALS Airborne Coastal Mapping: Past, Present, and Future. Journal of Coastal Research 38(SPEC. ISS), pp. 207–215.
Wozencraft, J. and Millar, D. (2005). Airborne LiDAR and integrated technologies for coastal mapping and nautical charting. Marine Technology Society Journal 39(3), 27–35. https://www.ingentaconnect.com/content/mts/mtsj/2005/00000039/00000003/art00003
Wright, C. W., Kranenburg, C., Battista, T. A. and Parrish, C. (2016). Depth Calibration and Validation of the Experimental Advanced Airborne Research LiDAR, EAARL-B. Journal of Coastal Research.
Wu, C. (2022). VisualSFM : A Visual Structure from Motion System. http://ccwu.me/vsfm
Wu, Z., Mao, Z., Shen, W., Yuan, D., Zhang, X. and Huang, H. (2022). Satellite-derived bathymetry based on machine learning models and an updated quasi-analytical algorithm approach. Opt. Express 30(10), pp. 16773–16793. http://opg.optica.org/oe/abstract.cfm?URI=oe-30-10-16773
Xu, J., Zhou, G., Su, S., Cao, Q. and Tian, Z. (2022). The development of a rigorous model for bathymetric mapping from multispectral satellite-images. Remote Sensing 14(10). https://www.mdpi.com/2072-4292/14/10/2495
Xu, W., Guo, K., Liu, Y., Tian, Z., Tang, Q., Dong, Z. and Li, J. (2021). Refraction error correction of Airborne LiDAR Bathymetry data considering sea surface waves. International Journal of Applied Earth Observation and Geoinformation 102, 102402. https://www.sciencedirect.com/science/article/pii/S0303243421001094
Xu, W., Zhang, F., Jiang, T., Feng, Y., Liu, Y., Dong, Z. and Tang, Q. (2022). Feature curve-based registration for airborne LiDAR bathymetry point clouds. International Journal of Applied Earth Observation and Geoinformation 112, 102883. https://www.sciencedirect.com/science/article/pii/S1569843222000851
Xue, Q., Sun, Q., Wang, F., Bai, H., Yang, B. and Li, Q. (2021). Underwater High-Precision 3D Reconstruction System Based on Rotating Scanning. Sensors 21(4). https://www.mdpi.com/1424-8220/21/4/1402
Yang, F., Qi, C., Su, D., Ding, S., He, Y. and Ma, Y. (2022). An airborne LiDAR bathymetric waveform decomposition method in very shallow water: A case study around Yuanzhi island in the South china sea. International Journal of Applied Earth Observation and Geoinformation 109, 102788. https://www.sciencedirect.com/science/article/pii/S0303243422001143
Yang, F., Su, D., Ma, Y., Feng, C., Yang, A. and Wang, M. (2017). Refraction Correction of Airborne LiDAR Bathymetry Based on Sea Surface Profile and Ray Tracing. IEEE Transactions on Geoscience and Remote Sensing 55(11), pp. 6141–6149.
Yang, H., Ju, J., Guo, H., Qiao, B., Nie, B. and Zhu, L. (2022). Bathymetric Inversion and Mapping of Two Shallow Lakes Using Sentinel-2 Imagery and Bathymetry Data in the Central Tibetan Plateau. IEEE Journal of Selected Topics in Applied Earth Observations and Remote Sensing 15, pp. 4279–4296.
Yang, Z., Yu, X., Dedman, S., Rosso, M., Zhu, J., Yang, J., Xia, Y., Tian, Y., Zhang, G. and Wang, J. (2022). UAV remote sensing applications in marine monitoring: Knowledge visualization and review. Science of The Total Environment 838, 155939. https://www.sciencedirect.com/science/article/pii/S0048969722030364
Zhang, H., Wang, J., Li, D., Fu, B., Lou, X. and Wu, Z. (2022). Reconstruction of large complex sand-wave bathymetry with adaptive partitioning combining satellite imagery and sparse multi-beam data. Journal of Oceanology and Limnology 2523-3521. https://doi.org/10.1007/s00343-021-1216-5
Zhang, X., Chen, Y., Le, Y., Zhang, D., Yan, Q., Dong, Y., Han, W. and Wang, L. (2022). Nearshore Bathymetry Based on ICESat-2 and Multispectral Images: Comparison Between Sentinel-2, Landsat-8, and Testing Gaofen-2. IEEE Journal of Selected Topics in Applied Earth Observations and Remote Sensing 15, 2449–2462.
Zhang, Z., Chen, P. and Mao, Z. (2022). Sols: An open-source spaceborne oceanic LiDAR simulator. Remote Sensing 14(8). https://www.mdpi.com/2072-4292/14/8/1849
Zhao, X., Tian, X., Wang, H., Liu, Q. and Liang, S. (2012). Chapter 5 – Atmospheric Correction of Optical Imagery. S. Liang, X. Li and J. Wang, eds, Advanced Remote Sensing. Academic Press, Boston, pp. 111–126. https://www.sciencedirect.com/science/article/pii/B9780123859549000058
Zhao, X., Xia, H., Zhao, J. and Zhou, F. (2022). Adaptive wavelet threshold denoising for bathymetric laser full-waveforms with weak bottom returns. IEEE Geoscience and Remote Sensing Letters 19, pp. 1–5.
Zhao, X., Zhao, J., Zhang, H. and Zhou, F. (2018). Remote Sensing of Sub-Surface Suspended Sediment Concentration by Using the Range Bias of Green Surface Point of Airborne LiDAR Bathymetry. Remote Sensing 10(5). https://www.mdpi.com/2072-4292/10/5/681
Zheng, H., Ma, Y., Huang, J., Yang, J., Su, D., Yang, F. and Wang, X. H. (2022). Deriving vertical profiles of chlorophyll-a concentration in the upper layer of seawaters using ICESat-2 photon-counting LiDAR. Opt. Express 30(18), 33320–33336. http://opg.optica.org/oe/abstract.cfm?URI=oe-30-18-33320
Zhou, D.-X. (2020). Universality of deep convolutional neural networks. Applied and Computational Harmonic Analysis 48(2), pp. 787–794.
Zhou, Y., Lu, L., Li, L., Zhang, Q. and Zhang, P. (2021). A generic method to derive coastal bathymetry from satellite photogrammetry for tsunami hazard assessment. Geophysical Research Letters 48(21), e2021GL095142. e2021GL095142 2021GL095142. https://agupubs.onlinelibrary.wiley.com/doi/abs/10.1029/2021GL095142
Zou, L., Qi, Y. and Wang, G. (2016). A method of image-based water surface reconstruction. J. J. J. H. Park, H. Jin, Y.-S. Jeong and M. K. Khan, eds, Advanced Multimedia and Ubiquitous Engineering. Springer Singapore, Singapore, pp. 437–443.
9. AUTHOR BIOGRAPHY
Assoc. Prof. Dr. Gottfried Mandlburger studied geodesy at Technische Universität Wien, where he also received his PhD in 2006 and habilitated in photogrammetry with a thesis on “Bathymetry from active and passive photogrammetry”. In March 2022 he was appointed Assistant Professor. His main research areas are airborne topographic and bathymetric LiDAR from crewed and uncrewed platforms, multimedia photogrammetry, bathymetry from multispectral images, and scientific software development. Gottfried Mandlburger is chair of the DGPF working group hydrography/bathymetry and received best paper awards from ISPRS (2019) and ASPRS (2019) for recent publications on bathymetry from active and passive photogrammetry. Email: gottfried.mandlburger@geo.tuwien.ac.at