Abstract
1 Introduction
The ocean is everything to us: life, food, energy, recreation and jobs. Right now, the ocean is under pressure and threatened by overexploitation, loss of biodiversity and habitats, pollution, marine littering and climate change. The value of the ocean economy speaks to its importance: The Organization for Economic Cooperation and Development (OECD) estimates that by 2030, $3 trillion USD will be generated annually from ocean sectors such as transportation, fishing, tourism, and energy (Pendleton et al., 2020). But the ocean and in particular the coastal regions are rapidly changing, and it is not entirely clear how these changes will play out. At the same time, the ocean is increasingly understood as essential for achieving sustainable development, including climate and biodiversity goals (Gaill et al., 2022; Bender et al., 2022).
Digital Twins of The Ocean or Digital Ocean Twins set out to bring relevant ocean data and information from different sources into new contexts (Fig. 1), to understand relations that are crucial for society to develop sustainable economic activities in the ocean. Digital Twins for climate change scenarios, in complement to traditional climate models, provide the knowledge to further understand the ocean’s relation with Earth’s changing climate, to understand anthropogenic impacts, and empower scientists, politicians and ocean stakeholders with the necessary information for evidence-based decision-making for sustainable management of the Ocean and adaptation to climate change (Larkin et al., 2021). In addition, data are increasingly important to meet the ambitious targets set out in the European Green Deal, and its related Climate Pact, pledging for Europe to be climate neutral by 2050 (EU Commission, 2019).
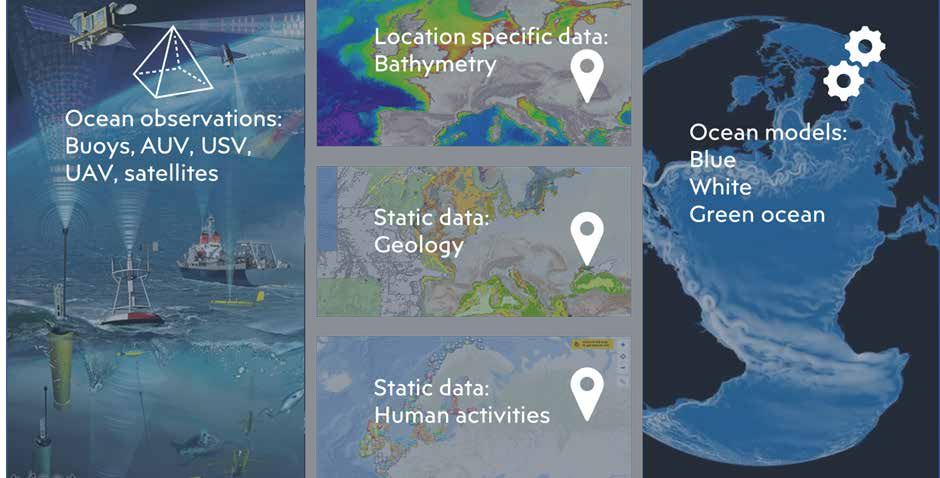
Digital Twins are famously used in the manufacturing and asset heavy industries and have made it into the complex fields like urban planning, or optimization of supply chain and other operational processes lately. Digitalisation and the use of digital technologies within the marine and maritime industry offer enormous scaling potential in both economic and environmental terms (Biber et al., 2022).
Knowledge of the ocean as a dynamical entity with predictable features, for example the regularity of its currents and tides and seasonal variations have been known for millennia. Knowledge of oceanography likely helped the successful colonization of Oceania, and similarly Viking and Inuit navigation, the oldest known dock was constructed with knowledge of the tides dating back to 2500–1500 BCE, and in the 8th century CE the existence of tides was correctly attributed to the Moon’s pull (Sonnewald et al., 2021a). In the last century, several institutes have started to compile central repositories with ocean data from ocean observations and ocean models, but still there are only a few applications that analyse data in the context of cross-domain activities. The explosion of data in the last few years with the advent of the fourth industrial revolution and its technologies Internet of things, Big data, Artificial Intelligence and Machine Learning as well as Digital Twins meant a shift towards the integration of diverse data from different data sources and the need for a step change in geospatial and ocean data information systems to move beyond ‘data’ as a focal point of activity, to processing and synthesising data into contextualized information, so that it can be readily used to gain new knowledge and insights (UN-GGIM, 2022).
Sustainable development of the ocean refers to the management and use of ocean resources in a way that meets the needs of the present generation without compromising the ability of future generations to meet their own needs. This often involves multiple stakeholders, including government agencies, fishing communities, environmental organisations, and corporations, who have competing interests and goals.
Digitalization, particularly through the use of digital twins of the ocean, can play a significant role in promoting sustainable development of the ocean (Hassani et al., 2022; Biber et al., 2022). A digital twin is a virtual representation of a real-world system, such as the ocean, that can be used to analyse and simulate various scenarios and outcomes. In the context of ocean sustainability, digital twins can provide valuable insights into the current state and future projections of ocean ecosystems and the human activities that impact them. This information can be used to inform decision-making and management strategies that promote sustainable use of ocean resources. Additionally, digital twins can support the coexistence of multiple stakeholders in the same ocean space by providing a shared platform for data-driven decision-making and collaboration (Senter for hav og Arktis, 2022).
By creating a virtual representation of the ocean that can be accessed and analysed by multiple stakeholders, digital twins can help to facilitate communication and collaboration among government agencies, ocean industries, and environmental organisations, as well as support the development of more equitable and sustainable ocean management policies.
In this work, we are introducing the terms Digital Twins of the Ocean (DTO), digital twins that look at the ocean and its importance for climate and biodiversity, and Digital Ocean Twins (DOT), digital twins of the ocean combined with twins of human activity in the ocean, like marine industries, tourism and maritime transport. Like DTO, DOT will be tailored to specific scientific questions or application that look at the ocean system for marine spatial planning, ocean protection and development of a sustainable blue economy.
We are giving examples of DTO and DOT that are already existing or under development and highlight their importance and purpose within ocean science and ocean use.
2 Digital twins of the ocean – a revolution for science and ocean governance
Digital Twins of the Ocean or Digital Ocean Twins will revolutionise ocean science, oceanography, and hydrography as we know it.
Three characteristics of Digital Twins will drive this revolution:
- Data contextualisation
- AI-driven data science and analytics
- New ways of interacting with and presentation of data
Definitions (see also Object Management Group, 2020)
Digital Twins are a virtual replica of the physical world. Changes in the real system are reflected in the digital twin and the twin can be used to modify the real system (feedback).
Digital Twins of the Ocean (DTO) are virtual representations of the ocean with its physical, chemical and biological properties, based on ocean observations and ocean models with the purpose of what-if scenarios for decision making. The feedback of DTO is the implementation of decisions based on the DTO.
Digital Ocean Twins (DOT) are the combination of DTO with digital twins of assets and activities in the ocean for purpose to study their interaction and importance for operational decision making, protection of the marine environment and a sustainable ocean economy that includes marine spatial planning and marine protected areas. The feedback of DOT can include operational changes in (near) real-time triggered by events in the twin for optimisation towards economic and environmental goals.
Both, DTO and DOT are developed for specific purposes and for specific ocean areas (from the global ocean to local areas of interest). This implies that the T in DTO and DOT will always be in the plural form, and some will be a system of interoperable twins.
Asset-driven have already been developed and used for years and are focused on specific assets or components assets (GISGRO, 2023; Fig. 2).

Asset twins can be applied to the ocean domain for ships, fishing gear, wind parks or oil platforms. They provide detailed information on the performance and behaviour of these assets and are typically used to optimize operations and improve efficiency, e.g. through predictive maintenance.
Process-driven twins have been applied successfully in cities for logistics and in production values chains (Jiang, 2021). They can be applied to the ocean domain for ship routing, port logistics or aquaculture operations. The main difference between these twins and DTO/DOT is the geospatial domain. DTO/DOT as geospatial twins include physical location, geography, hydrological and oceanographic information and their associated environmental, social, and economic factors to consider the interconnections between ocean systems and human activities.
3 Data contextualisation
The power of digital twins is their AI-supported ability to combine data from different sources and analyse them in context, often to reveal connections that were unknown before. ESA’s Digital Twin Hydrology combines data from satellite and ground observations with model results in a warning system for landslides and flooding (ESA, 2021).
The infographic in Fig. 3 visualises this.

Ocean observation systems are developed and managed all over the world. Programs like the global ocean observing system GOOS, deliver a permanent global system for observations, modelling, and analysis of marine and ocean data (GOOS, 2021; EuroGOOS, 2023; ODYSSEA, 2021; NorEmso, 2021; OceanLab, 2023).
Ocean observations play a crucial role in the development of digital twins of the ocean. They provide the data necessary to create an accurate digital twin. Observations can come from various sources, including satellite imagery, localised in situ measurements (Fig.4), and mobile sensors on autonomous systems. Each observation has a specific range and purpose and combined, they provide data for an ocean area from space to seabed, also called the observation pyramid (NTNU, 2022).
The combination of real-time observations creates a comprehensive picture of the ocean’s current state and time series help to identify patterns and trends over time.
Data from ocean observatories can be assimilated into ocean models to improve their quality (Fig. 5). An ocean model is a mathematical description of the ocean’s physical, chemical, and biological processes. This is also referred to as the blue, white, and green ocean.
Ocean models can describe the ocean state in the past, present and future and results are hindcasts (reanalyses, with assimilated ocean observations), nowcasts (analyses), and forecasts (prediction of future states) (CMEMS, 2021).
Ocean models provide a comprehensive representation of the ocean’s state and its evolution over time over a large area and period which has some advantages over ocean observations that cover either a specific location or time. By varying the socalled boundary conditions, which describe the ‘outside’ world, ocean models can be used to simulate so-called what-if scenarios, an important aspect of digital twins. Scenarios that take into account climate change, extreme weather events or changes to the seafloor or coastal infrastructure and their effects are examples of what-if scenarios. Ocean models can be combined with ecosystem models to assess opportunities and challenges for biomass production, to predict threats like algae blooms, parasites or infections or water quality Digital parameters like temperature, oxygen and nutrition levels. Environmental modelling combines ocean and ecosystem models to understand the natural systems and how they react to acute changes, such as exposure to pollution (noise, litter, hazardous substances) and the temporal and dose effects from this exposure.
Digital Twins of the Ocean will employ models in different spatial and time resolution depending on the purpose of the digital twin. A digital twin for environmental modelling in a fjord has high requirements to resolution as a digital twin that is developed to assess climate change scenarios. In contrast to digital solutions that we know from before, operational DOT will support management and mitigation through more accurate information and recommendations.


4 AI-driven data science and analytics in digital twins
Digital Twins of the Ocean by their nature come with a set of challenges that Artificial Intelligence (AI) and its subcategory Machine Learning (ML) can help address (Google, 2023):
- AI allows a machine to simulate human intelligence to solve problems.
- AI uses technologies to mimic human decision-making.
- AI systems use logic and decision trees to learn and self-correct.
- ML allows a machine to learn autonomously from past data.
- ML uses self-learning algorithms to produce predictive models.
While the adoption of AI and ML within digital twins is not without risk, they are increasingly being recognized as tools that can automate tasks and perform them more reliable and faster, enhance what can be learned from different forms of observations and their comparison with model results, and generate actionable insights as (Sonnewald et al., 2021a; Fig. 6).
Data fusion and analysis: Observational data is often spatially sparse, limited to the ocean’s surface, and few time series span decades. Unfortunately, important timescales span seconds to millennia in the real ocean, with strong scale interactions which complicate numerical modelling together with details such as coastlines and islands (Sonnewald et al., 2020; Sonnewald et al. 2021b). ML shows the potential to bridge and fill the gaps in our data record, for example by finding and predicting patterns..
Predictions: A basic goal and a test of the understanding of a process is the ability to predict its behaviour. ML has the power to enhance predictions beyond a few days through pattern recognition and time series analysis.
Enhanced quality and efficiency: ML can improve and enhance ocean modelling especially on edge (remote) devices with less computational power like mobile sensor platforms or offshore observatories. ML methods will enable the efficient use of hardware and advance processing on edge devices even further (Wehner et al., 2022).
Decision support: As digital twins use datasets from different data sources, on different reference grids and for different variables, these data can be fused and compared using ML techniques. ML can also be used for the quantification of uncertainties within the data and from these processes. This can be accounted for when data is presented for decision making and recommendations (Sonnewanld et al. 2021).
In oceanography, hydrography and their application in digital twins, ML can enhance data exploration. Where traditional approaches to data analysis ground their data in prior knowledge and assumptions, a data-exploration centred approach allows the possibility of serendipitous discoveries and can unveil previously unknown relations and dependencies.
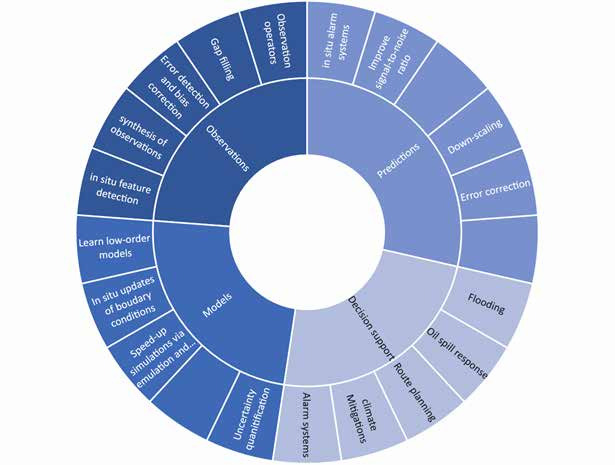
5 Interacting with digital ocean twins
Digital ocean twins have the ambition to open up to new fields of applications and presentations for their data, connecting oceanography and hydrography with new industries, like gaming and other immersive 3D digital environments. Initiatives like Worlding (MIT, 2022) or Immerse (Immerse, 2023) use Digital twinning for engaging storytelling and ideation between participants. Game engine industry companies like Unity and Unreal engage with digital twin communities for powerful visualisations. Digital twinning is a growth market for the game engine industry, in verticals such as manufacturing, architecture, finance, and medicine but also simulation and training for ocean industries. “Digital twinning gives teams the power to ideate”, said Elizabeth Baron, a senior manager of enterprise solutions at Unity in her talk at WORLDING. “You can look at many things that maybe aren’t even possible to produce. But you’re the resource. Impact is very low, but the creativity aspect is very high”.
The Maritime Spatial Planning Challenge (MSP Challenge, 2023) is a next generation planning support game that also features a virtual and augmented reality module called Ocean View. The developers are a partner in the EU funded Digital Twins of the Ocean project Iliad (Iliad, 2022), where they aim at further development and linking the platform to the project’s Digital Twins of The Ocean. At the International Digital Twins of the Ocean summit (G7FSOI, 2022), presentations featured powerful visualisations by the Unreal gaming engine which used ocean data and weather forecasts for storytelling by The Weather Channel in the US (Epic Games, 2023).
These kinds of visualisations have the potential to play a significant role in promoting sustainable development of the ocean. They allow stakeholders to interact with and explore virtual representations of ocean systems and the impacts of human activities on these systems in a way that is immersive and engaging:
- Stakeholder engagement: virtual environments where stakeholders, including the public, policy makers, and industry leaders, can interact with and explore the ocean in an engaging way can raise awareness about the importance of sustainable ocean development and encourage action.
- Environmental education: Interactive visualisations can be used to inform people about the ocean, its biodiversity, and the impacts of human activities on these systems. This can help build a greater understanding of the importance of sustainable ocean development and the need for action.
- Decision-making support: Visualisations can be used to support decision-making by allowing stakeholders to explore scenarios and the impacts of different management strategies on ocean systems for more effective and sustainable management including protection of ocean areas.
- Collaborative planning: engaging visualisations can be used to create virtual environments where stakeholders can collaborate on solutions to ocean sustainability challenges. This can foster greater understanding and cooperation among stakeholders that want to operate in the same ocean area.
Researchers do often have different needs to data visualisation as they want to understand relations between data and phenomena and drill down into the data. They will need another type of interactive visualisation to cater to those needs. DTO/DOT will deliver visualisations in the form of interactive dashboards (e.g. Grafana, which is tailored to ocean observations, (SINTEF Ocean, 2023) or embedded in jupyter notebooks with interactive libraries like Plotly (Plotly, 2023), Bokeh (Bokeh, 2022) or Holoviews (Holoviews, 2023) based on accurate and up-to-date data that include clear objectives and an understanding of their assumptions and limitations.
6 Data and twin interoperability
Data contextualisation, AI-driven data science and analytics and the presented ways of interacting with data within digital twins come with ambitious requirements for data and twin interoperability.
The FAIR Data Principles define the properties of data objects to ensure that data are reusable by humans, machines, and applications like digital twins: Findable, Accessible, Interoperable, and Reusable (Lin et al., 2020; Fig. 7).
FAIR principles are accompanied by an endorsed set of guiding principles to demonstrate trustworthiness of the data repositories to the user of the data: Transparency, Responsibility, User focus, Sustainability and Technology – TRUST (Fig. 8).
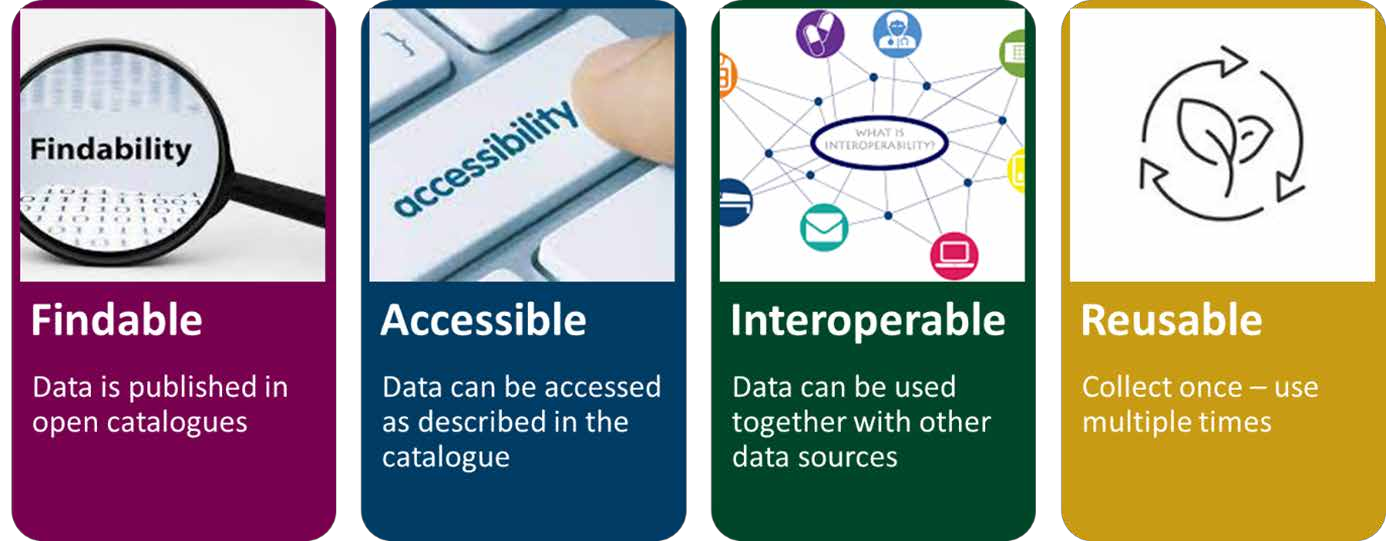
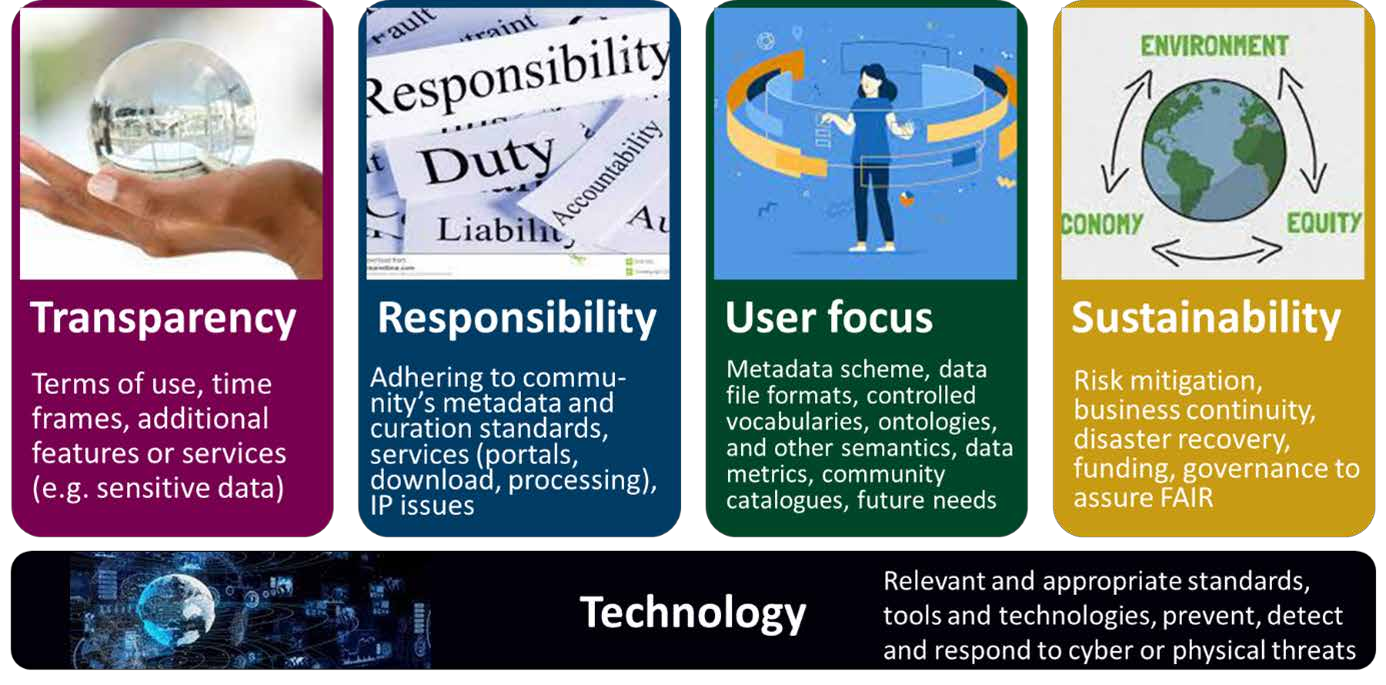
Digital twins require these principles to apply without the human in the loop, i.e. through defined standards and application programming interfaces (API) that ensure machine to machine (M2M) findability, accessibility, interoperability and reusability. The United Nations Decade of Ocean Science for sustainable development has defined interoperable ocean data as one of the necessary building blocks to address the identified challenges and has implemented a Data Coordination Group to provide advice to Decade coordination and governance structures (IOC-UNESCO, 2021) “The ambition of the Decade in relation to data, information and knowledge management includes significant enhancement of infrastructure, common approaches that enable interoperable data sharing and stewardship, and enhanced collaboration between data”. The group is accompanied by a technical group for implementation, a corporate data group to unlock data from the private sector on the same grounds and the Decade Coordination Office (DCO) for Ocean Data Sharing.
The UN Decade program DITTO – Digital Twins of the Ocean (DITTO, 2022; Fig. 9) as well as the associated program TURTLE – Interoperability architectures for Digital Twins of the Ocean (Brönner et al., 2022) have been established to gather Digital Twin communities across the globe to promote standards and building blocks for Digital Twins of the Ocean.

Semantics play a crucial role in data and digital twin interoperability. They describe the meaning of data and the relationships between different aspects of data. In the context of digital twins, semantics are used to describe the relationships between different data sources, modules or building block of twins and the relation between the data and the building block.
With semantic interoperability, different devices and systems can find, understand and interpret data and modules in the same way and in the context of digital twins, semantic interoperability is critical for ensuring that data from different sources can be integrated and analysed seamlessly.
7 How digital twins of the ocean advance sustainable ocean solutions
The objective of the Sustainable Blue Economy is to restore, protect and maintain the ocean with the help of technology that operates within the limits of our planet, while building a diverse, resilient and environmentally sustainable marine economy with social and economic benefits for current and future generations (Fig. 10).


Benefits from the use of digital twins of the ocean
The coastal area of our ocean is threatened by extreme weather events, storm surges and sea level rise caused by climate change. At the same time, our coasts become increasingly important as more and more people live in megacities at the coast. Ports need to ensure that they have the right infrastructure to supply these people with the foods and goods that they need. At the same time, infrastructure from offshore energy production like cables and pipelines arrive at the swash zone of the shore and are threatened by coastal erosion. Digital twins of the coastal ocean can help by informing decision making with what if-scenarios for coastal protection against coastal erosion as well as sediment transport for infrastructure protection while at the same time ensure biodiversity protection in infrastructure projects.


Benefits from the use of digital twins of the ocean
Digital twins of the ocean can be used in the planning phase to inform decision making with wind resource predictions, as well as geological and environmental information to find locations for sustainable development of wind parks. In the operation phase, DTO support noise, biodiversity and sea floor monitoring as well as predictive maintenance by fusing environmental twins with asset twins. They can also predict windows of opportunity for safe operations in the wind park from environmental conditions in the construction, operation and decommissioning phase.


Benefits from the use of digital twins of the ocean
Digital twin technology is already applied in aquaculture to monitor and optimize various aspects of fish farming operations like monitoring water quality parameters such as temperature, salinity, pH, and dissolved oxygen levels, which are crucial factors that can affect the health, welfare and biomass development of fish. Another application is the prediction for windows of operations as well as potential risks to fish and infrastructure, like changes in water quality or extreme weather which can help to prevent spreading of diseases, loss of biomass or accidents. Optimised feeding, water management, and other aspects of operations can reduce feed waste, costs and environmental impacts and hence improve the sustainability of aquaculture operations.


Benefits from the use of digital twins of the ocean
DOT support maritime transport with ship routing according to environmental conditions to reduce emissions or prevent accidents in the port under harsh conditions. They can support a digital port with risk assessment for invasive species, sediment transport, weather conditions for port operations tailored to cargo or passenger transport. Autonomous vessels like ferries are depending on accurate environmental conditions in real-time and for the near future. Electric vessels depend on environmental conditions to predict the range and plan routes.


Benefits from the use of digital twins of the ocean
A combination of satellite data, precipitation, and irrigation together with sea levels and river run off can be used in a DOT for flash flood prediction and land slide warning. Digital modelling of the physical environment includes terrain, buildings, and bridges. This DOT can save human lives and economic damage from destruction of infrastructure in extreme flood events.


Benefits from the use of digital twins of the ocean
DOT for fisheries management combine data from fishing vessels on physical ocean data together with fuel consumption and catch data to optimise routing and reduce emissions from fishing fleets. If additionally catch and bycatch data are combined with stock assessments, the DOT can employ catch prediction models to support fish stock management and fisheries productivity while minimising the environmental footprint through optimisation of fishing activity and ecosystem-based management.

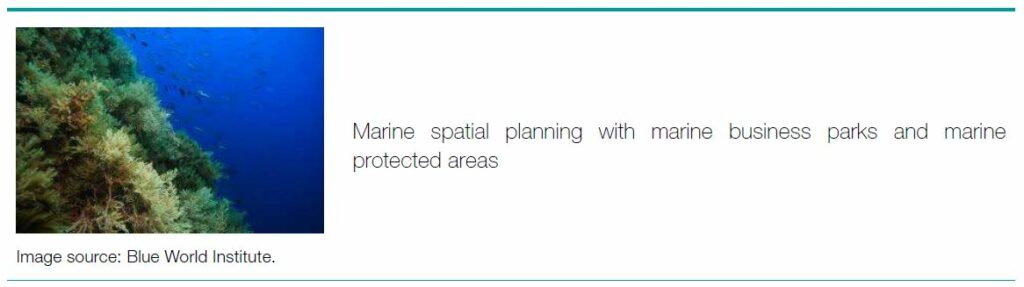
Benefits from the use of digital twins of the ocean
Marine protected areas (MPAs) are established for the conservation of their natural or cultural resources. While there can be restrictions on certain activities in MPAs, nearly all U.S. MPAs allow multiple uses, including fishing.
Marine business parks, with co-location of several activities in a limited area, will be able to reduce the total area use and form the basis for efficient resource utilization outside of MPAs. Co-operation will be able to utilize infrastructure more efficiently, and reduce costs for both infrastructure, operation, monitoring and preparedness. Great opportunities for data sharing and digitalisation will also reduce costs and at the same time provide a better basis for decision-making than today for both industry and administration. A virtual pilot project (“digital twin”) with relevant industry players and other stakeholders can assess the possibilities and gains from establishing a marine business park through a set of what if scenarios.

8 Conclusion
Digital Twins of the Ocean or Digital Ocean Twins have great potential to revolutionise ocean science, oceanography, and hydrography as we know it through data contextualisation and new and better insights from combination of a variety of data sources. Artificial Intelligence for automation of data processes and Machine Learning for data analytics will be essential and support data fusion, provide data insight from pattern recognition and inform and support decision making through recommendations. Additionally, Digital Twins will rely on and support new ways of interacting with the data they are based on and which they produce. Digital twins are different from other digital ocean applications in that they implement a two-way data exchange and feedback loop where the twin changes according to the change in the real-world system and the real-world system changes according to changes in the twin. This can either be a change due to a decision based on twin simulations (what if scenarios), or it can be a direct change of the physical twin triggered by an event observed in the digital twin (alarms, automation, autonomous operations).
Through these capabilities, digital twins of the ocean can support and foster a sustainable blue economy that operates within the limits of nature. It can provide guidance and support on where to establish Marine Protected Areas as well as Marine Business Parks where different ocean industries are co-located to leverage synergies.
The International Hydrographic Organization (IHO) can play a key role in promoting the integration of hydrographic and oceanographic data to create more accurate and comprehensive digital twins of the ocean particularly through its work in establishing standards and best practices for hydrographic data collection and management. The combination of data from the fields of hydrography and oceanography can provide a better understanding of the ocean, improve ocean management, and support sustainable use of ocean resources specifically in the fields of shipping and coastal management.
Digital twins have very ambitious requirements to data availability, as well as data and twin interoperability. Digital Twins of the Ocean or Digital Ocean Twins will always be developed for a specific purpose and / or specific ocean area. This implies that several twins might be combined into a system of twins and need to be interoperable. To facilitate and leverage the capabilities of AI and ML for data fusion, analytics and predictions, data need to follow FAIR and TRUST principles for data availability. While there are several initiatives to promote these ambitions within the UN Decade for Ocean Science there is still a way to go before all data repositories follow these principles.
Digital Twins of the Ocean and Digital Ocean Twins provide powerful tools for ecosystem-based management of ocean industries, marine spatial planning and the development of Marine Protected Areas. They are thereby well-suited as a tool for political decisions in these areas. Governments must support national data management and the development of national data repositories and infrastructures for digital twins which have not been addressed explicitly in this paper.
Acknowledgements
Ute Brönner’s time was partly funded through the Iliad project that has received funding from the European Commission’s Horizon 2020 Research and Innovation programme under grant agreement No 101037643.
References
Bender M., Bustamante, R., and Leonard, K. (2022). Living in relationship with the Ocean to transform governance in the UN Ocean Decade. PLOS Biology, 20(10): e3001828. https://doi. org/10.1371/journal.pbio.3001828
Biber, A., Knodt, S., and Visbeck, M. (2022). Sustainable Blue Economy Transformation, Value and the Potential of Marine Ecosystems. https://www.feri.de/media/nmsjlhkk/fcfi_blue_economy.pdf
Bokeh (2022). Viewed online 12.03.2023: https://bokeh.org/ Brönner, U. et al. (2022). Interoperability ArchitecTURes for a DigiTaLOcEan (TURTLE). Viewed online 12.03.2023: https://forum.oceandecade.org/ventures/89109?p=true&ventures_venture=89109
Cagri Inan Alperen et al. (2021). A Hydrological Digital Twin by Artificial Neural Networks for Flood Simulation in Gardon de SainteCroix Basin. France IOP Conf. Ser.: Earth Environ. Sci. 906 012112.
CMEMS (2021). Ocean Monitoring and Forecasting Models. Viewed online 12.03.2023: https://marine.copernicus.eu/explainers/operational-oceanography/monitoring-forecasting/models.
Coetzee, S., Gould, M., McCormack, B., Zaffar Sadiq Mohamed-Ghouse, E., Vision, S., Greg Scott, A., and Alameh, N. (2021). Towards a sustainable geospatial ecosystem beyond SDIs. https://ggim.un.org/meetings/GGIM-committee/11th-Session/documents/Towards_a_Sustainable_Geospatial_Ecosystem_Beyond_SDIs_Draft_3Aug2021.pdf
DITTO (2022). DITTO – Digital Twins of the Ocean. DITTO a Global Program of the UN Decade of Ocean Science for Sustainable Development (2021–2030). Viewed online 12.03.2023: https://ditto-oceandecade.org/
Epic Games (2023). Viewed online 12.03.2023: https://www.unrealengine.com/en-US/spotlights/floods-and-fires-how-the-weather-channel-uses-unreal-engine-to-keep-you-safe
ESA (2023). Digital Twin Hydrology. The European Space Agency. Viewed online 12.03.2023: https://www.esa.int/ESA_Multimedia/ Videos/2021/10/Digital_Twin_Hydrology EU Comission (2019). Communication on The European Green Deal. Viewed online 12.03.2023: https://commission.europa.eu/publications/communication-european-green-deal_en
EURACTIV (2018). Viewed online 12.03.2023: https://www.euractiv.com/section/circular-economy/opinion/the-sustainable-blueeconomy-eus-actions-must-match-its-words/
EuroGOOS (2023). Viewed online 12.03.2023: https://eurogoos.eu/
G7FSOI (2022). International Digital Twins of the Ocean Summit, Session Presentations and Replays. Viewed online 12.03.2023:
Gaill, F., Brodie Rudolph, T., Lebleu, L. et al. (2022). An evolution towards scientific consensus for a sustainable ocean future. npj Ocean Sustain, 1(7). https://doi.org/10.1038/s44183-022-00007
GISGRO (2023). Viewed online 12.03.2023: https://www.gisgro. com/digital-twin-in-asset-management/
Google (2023). Viewed online 12.03.2023: https://cloud.google. com/learn/artificial-intelligence-vs-machine-learning
GOOS (2021). The Global Ocean Observation System. Viewed online 12.03.2023: https://www.goosocean.org/
Hassani, H., Huang, X., and MacFeely, S. (2022). Enabling Digital Twins to Support the UN SDGs. Big Data and Cognitive Computing, 6(4), p.115.
Holoviews (2023). Viewed online 12.03.2023: https://holoviews.org/reference/
Iliad (2022). IliadDigital Twin of the Ocean. European Commission’s Horizon 2020 Research and Innovation programme. Viewed online 12.03.2023: https://www.ocean-twin.eu/
Immerse (2023). Viewed online 12.03.2023: https://immerse.news/
IOC-UNESCO (2021). Data Coordination Group. Viewed online 12.03.2023: https://oceandecade.org/news/newly-appointedgroup-of-global-experts-will-help-drive-ocean-decade-actionon-data-information-and-knowledge-management/
Jiang Y, Yin S, Li K, Luo H, and Kaynak O. (2021). Industrial applications of digital twins. Philos Trans A Math Phys Eng Sci, 379(2207):20200360. https://doi.org/10.1098/rsta.2020.0360
Larkin, K. E., Marsan, A. A., Tonné, N., van Isacker, N., Collart, T., Delaney, C., Vasquez, M., Manca, E., Lillis, H., and Calewaert, J. B. (2021). Connecting marine data to society. Ocean Science Data: Collection, Management, Networking and Services. https://doi.org/10.1016/B978-0-12-823427-3.00003-7
Lin, D., Crabtree, J., Dillo, I. et al. (2020). The TRUST Principles for digital repositories. Sci Data 7(144). https://doi.org/10.1038/ s41597-020-0486-7
MIT (2022). WORLDING. Viewed online 12.03.2023: https://news.mit.edu/2022/worlding-game-engines-twins-climate-futures-stories-1201
MSPChallenge (2023). Maritime Spatial Planning Challenge. Viewed online 12.03.2023: https://www.mspchallenge.info/
NorEmso (2021). Viewed online 12.03.2023: https://www.uib.no/en/noremso
NTNU (2022). The observation pyramid. Viewed online 12.03.2023: https://www.youtube.com/watch?v=K_LKkiL1RTc
Object Management Group (2020). Viewed online 12.03.2023 https://www.digitaltwinconsortium.org/Initiatives/the-Definition- of-a-Digital-Twin/
OceanLab (2023). Viewed online 12.03.2023: https://www.ntnu.edu/oceanlab
ODYSSEA (2021). Viewed online 12.03.2023: https://odysseaplatform.eu/
Pendleton L., Evans K., and Visbeck M. (2020). We need a global movement to transform ocean science for a better world. https:// doi.org/10.1073/pnas.2005485117
Plotly (2023). Viewed online 12.03.2023: https://plotly.com/python/ipython-notebook-tutorial/
Senter for hav og Arktis (2022). Marine næringsparker – nye muligheter for samhandling til havs. (Norwegian)
SINTEF Ocean (2023). OceanLab Observatory. Viewed online 12.03.2023: https://oceanlab.azure.sintef.no/, https://grafana.com/
Sonnewald, M., Dutkiewitz, S., Hill, C., and Forget, G. (2020). Elucidating Ecological Complexity: Unsupervised Learning determines global marine eco-provinces, Science Advances. https://advances.sciencemag.org/content/6/22/eaay4740
Sonnewald, M., Lguensat, R., Jones, D. C., Dueben, P. D., Brajard, J., and Balaji, V. (2021a). Bridging observations, theory and numerical simulation of the ocean using machine learning. Environmental Research Letters, 16(7). https://doi.org/10.1088/1748-9326/ac0eb0
Sonnewald, M., and Lguensat, R. (2021). Revealing the impact of global warming on climate modes using transparent machine learning. Journal of Advances in Modeling Earth Systems. https://agupubs.onlinelibrary.wiley.com/doi/full/10.1029/2021MS002496
UN-GGIM (2022). United Nations Committee of Experts on Global Geospatial Information Management. Future Geospatial Information Ecosystem: From SDI to SoS and on to the Geoverse Making the Step Change Using the Integrated Geospatial Information Framework. https://ggim.un.org/meetings/GGIM-committee/12th-Session/documents/Future_Geospatial_Information_Ecosystem_Discussion_Paper_July2022.pdf
Verboven, P., Defraeye, T., Datta, A. K., and Nicolai, B. (2020). Digital twins of food process operations: the next step for food process models? Current Opinion in Food Science, 35, 79–87. https://doi.org/10.1016/j.cofs.2020.03.002
Wehner, D., Dell, S., Neumann, A. J., Wendt, J. (2022). MARISPACE-X: Digitalizing the ocean. The International Hydrographic Review, 28, 76–93. https://doi.org/10.58440/ihr-28-a10
Authors’ biographies

Ute Brönner is an ocean data enthusiast, passionate about understanding the ocean environment through data from the combination of sensor technology and modelling. She is a member of the Ocean Data Coordination Group of the UN Decade and the work package manager for pilots and demonstrators in the EU Horizon 2020 Digital Twins of the Ocean project Iliad. Together with Martin Visbeck she gathers Digital Twin of the Ocean communities in TURTLE, a UN Decade project on digital twin interoperability architectures. Her day-to-day job is as a senior project manager at SINTEF Ocean in Trondheim (Norway) where she works in the department for climate and environment on environmental modelling and environmental risk assessment, sustainable solutions for ocean industries, marine technology and the digitalisation of the oceans.

Maike Sonnewald is an Associate Research Scholar at Princeton University, an Affiliate Assistant Professor at the University of Washington and an Associate Researcher at the NOAA Geophysical Fluid Dynamics Laboratory. Focused on the ocean and climate, she has pioneered methods for physics-informed models, automated regime identification, and trustworthy machine learning applications. The influence of her work spans national and international policy, fundamental scientific discovery, and climate model development. Her work was highlighted in the NOAA Artificial Intelligence strategy (2021–2025) and informed the basis for New Zealand’s Marine Protected Area legislation. Her review articles include an invited contribution on machine learning applications in oceanography. She serves as Associate Editor at the journal Artificial Intelligence for the Earth System (American Meteorological Society).

Martin Visbeck holds the Chair in Physical Oceanography, GEOMAR Helmholtz-Centre for ocean research in Kiel and Kiel University, Germany. Martin Visbeck’s research is concerned with ocean and climate variability and change and ocean sustainability. He is developing new conceptual frameworks to advance integrated marine research in the context of ocean sustainable development at the regional and international level. He has contributed to ocean literacy projects and capacity building in Africa. His advice is thought by science bodies and governments in Germany, the EU and at the UN level. He is active in the governing board of the International Science Council (ISC), the leadership council of the Sustainable Development Solutions Network (SDSN) and the Executive Planning Group for the UN Decade of Ocean Science for Sustainable Development (2021–2030) as well as the UN Decade Program DITTO – Digital Twins of the Ocean.